![]() |
J Circ Biomark 2021; 10(1): 1-8 ISSN 1849-4544 | DOI: 10.33393/jcb.2021.2194 ORIGINAL RESEARCH ARTICLE |
![]() |
Value of clinical laboratory test for early prediction of mortality in patients with COVID-19: the BGM score
ABSTRACT
Background: COVID-19 causes high mortality and long hospitalization periods. The aim of this study was to search for new early prognostic strategies accessible to most health care centers.
Methods: Laboratory results, demographic and clinical data from 500 patients with positive SARS-CoV-2 infection were included in our study. The data set was split into training and test set prior to generating different multivariate models considering the occurrence of death as the response variable. A final computational method called the BGM score was obtained by combining the previous models and is available as an interactive web application.
Results: The logistic regression model comprising age, creatinine (CREA), D-dimer (DD), C-reactive protein (CRP), platelet count (PLT), and troponin I (TNI) showed a sensitivity of 47.3%, a specificity of 98.7%, a kappa of 0.56, and a balanced accuracy of 0.73. The CART classification tree yielded TNI, age, DD, and CRP as the most potent early predictors of mortality (sensitivity = 68.4%, specificity = 92.5%, kappa = 0.61, and balanced accuracy = 0.80). The artificial neural network including age, CREA, DD, CRP, PLT, and TNI yielded a sensitivity of 66.7%, a specificity of 92.3%, a kappa of 0.54, and a balanced accuracy of 0.79. Finally, the BGM score surpassed the prediction accuracy performance of the independent multivariate models, yielding a sensitivity of 73.7%, a specificity of 96.5%, a kappa of 0.74, and a balanced accuracy of 0.85.
Conclusions: The BGM score may support clinicians in managing COVID-19 patients and providing focused interventions to those with an increased risk of mortality.
Keywords: BGM score, Clinical biochemistry, COVID-19, Mortality prediction, Risk score, Serum biomarkers
Received: October 26, 2020
Accepted: December 15, 2020
Published online: February 8, 2021
This article includes supplementary material
Journal of Circulating Biomarkers - ISSN 1849-4544 - www.aboutscience.eu/jcb
© 2021 The Authors. This article is published by AboutScience and licensed under Creative Commons Attribution-NonCommercial 4.0 International (CC BY-NC 4.0).
Commercial use is not permitted and is subject to Publisher’s permissions. Full information is available at www.aboutscience.eu
Introduction
The SARS-CoV-2 virus emerged in the last quarter of 2019 in Wuhan, the capital of Hubei province of China. The disease caused by SARS-CoV-2 virus, named COVID-19 by the World Health Organization, has spread rapidly and globally causing a pandemic with unprecedented clinical, humanitarian, and economic repercussions (1,2). In the absence of reliable data on worldwide seroprevalence, the number of confirmed infections and deaths exceed 17.8 million and 680,000, respectively. The first studies that analyzed the clinical complications associated with this disease were from China. In this area, many of the patients had mild to moderate symptoms (80%), about 14% had a severe disease course (dyspnea, O2 saturation ≤93%, pulmonary infiltrates), and about 6% presented with critical progression characterized by respiratory failure, septic shock, and/or multiorgan failure (3). The data accumulated so far from more than 10,000 patients in the European Union and in the New York City area show that among the confirmed cases, 30% required admission and 4% required care in intensive care units (ICUs) due to their critical condition (4,5). In turn, it was observed that mortality is particularly high in the subgroup of patients with advanced age and preexisting comorbidities, among which obesity, hypertension, and diabetes are frequently found (6). It is noteworthy that patients without these associated comorbidities can also present with a critical or severe course of the disease. Therefore, the search for early biomarkers to assess the severity of the pathology and its clinical progression is currently necessary to rationalize the use of hospital resources in ICUs and reduce the mortality associated with COVID-19.
Several clinical laboratory markers, such as lymphocyte (LYMPH) count, lactate dehydrogenase (LDH), and D-dimer (DD), are altered in patients with COVID-19 (7). Other studies have shown significant differences in the concentration of cytokines in blood (interleukin [IL]-6, tumor necrosis factor [TNF]-γ, IL-8, IL-2R) among patients who have required ICU admission and patients who do not (8). In turn, infection biomarkers such as C-reactive protein (CRP), procalcitonin (PCT), and ferritin (FER) increased significantly with the severity of the disease (8). However, despite the research efforts made in the field of laboratory tests, reliable algorithms with early prognostic value have not yet been generated to establish the risk of future complications in patients infected with SARS-CoV-2.
The lack of accurate early prognostic algorithms based on central laboratory testing for COVID-19 has spurred researchers to direct their efforts toward the use of omics tools in the search for potential biomarkers. In a first study published by Shen et al (9), the combination of proteomics and metabolomics allowed the identification of a panel of 22 proteins and 7 metabolites with predictive power to differentiate mild vs. severe COVID-19 with 94% accuracy. A second study published in Cell Systems (10) showed that European researchers identified 27 differentially expressed proteomics biomarkers associated with different grades of COVID-19 severity in hospitalized patients. Despite these positive advances, the technical complexity of these omics tools and their high cost limit their applicability in the clinical arena.
In the context of the vast scope of the SARS-CoV-2 crisis and until we achieve sufficient immunization coverage of the population, we believe that the search for new early prognostic strategies must prioritize their applicability and accessibility to most health care centers. In this line, the objective of our study was to generate predictive algorithms for early stratification of patients with COVID-19 who may be at the risk of developing severe complications. To this aim, we designed a retrospective cross-sectional single-center study in which we evaluated different predictive algorithms for mortality considering demographic factors, clinical factors, and standard laboratory tests usually present in most central clinical laboratories.
Materials and methods
Patient population
Five hundred patients with COVID-19 confirmed by real-time reverse transcription polymerase chain reaction (RT-PCR) in nasopharyngeal exudates were included in this retrospective study. These patients required hospitalization in an ICU, internal medicine, or pneumology ward in our hospital between March and June 2020. The clinical and laboratory data that we collected in our database were the first information available within 48 hours after admission of the patient. Demographic and clinical data were obtained from our hospital information system (SAP Patient Management). The variables included were: age, sex, smoking and drinking habits, asthma, chronic obstructive pulmonary disease (COPD), diabetes mellitus, dyslipidemia, obesity, hypertension, heart failure, ischemic heart disease, hospitalization days, ICU stay, and in-hospital death. This study was approved by the Ethical Committee of the Hospital Clinic of Barcelona and was conducted following the ethical principles of the 1975 Declaration of Helsinki. The data set is available at the online repository figshare with DOI:10.6084/m9.figshare.13252277.
Laboratory measurements
Blood samples were collected in lithium heparin-, ethylenediaminetetraacetic acid-, and citrate-coated blood collection tubes for biochemical, hematological, and coagulation testing, respectively. After centrifugation at 3,000 rpm for 15 minutes, plasma samples were immediately processed. Alkaline phosphatase (ALP), alanine aminotransferase (ALT), aspartate aminotransferase (AST), total bilirubin (TBIL), creatinine (CREA), FER, gamma-glutamyl transferase (GGT), glucose (GLU), LDH, CRP, PCT, and troponin I (TNI) were measured using an Atellica Solution automated analyzer (Siemens Healthineers, Tarrytown, NY, USA). The intra-assay and inter-assay coefficient of variation was lower than 6% and 8%, respectively, in all cases. Hematological parameters (including hemoglobin [HB] and counts of white blood cells [WBC], neutrophils [NEU], LYMPH and platelets [PLT]) were analyzed without centrifugation using an Advia 2120 (Siemens Healthineers, Tarrytown, NY, USA). Finally, the Sysmex 5100 (Sysmex, Kobe, Japan) was used for DD, prothrombin time (PT), and partial thromboplastin time (PTT) analysis.
All the parameters were measured in the Core Laboratory of the Hospital Clinic of Barcelona according to the manufacturer’s instructions.
Statistical analysis
Categorical variables were expressed as numbers and percentages and compared using the Chi-square test. Continuous variables were expressed as median and interquartile range (IQR) and were compared by the Mann-Whitney-Wilcoxon test.
The strength of the relationship between the laboratory parameters was assessed using the Pearson or Spearman correlation coefficients. The multivariate statistical analyses conducted were logistic regression (LR) (11), classification tree (CT) through the CART algorithm (12), and artificial neural network (NNet) (13). Missing data were imputed via bagged tree models (11), and the data set was then split into a training and test set. The optimal parameter for each model was determined in the training set, calculating the best averaged predictive performance after 10-fold cross-validation. Additionally, to the previous multivariate models, we generated a computational method, called the BGM score, which provides the survival probability of a patient with COVID-19 considering the variables age, CREA, DD, CRP, PLT, and TNI. We modulated the survival probability of the BGM score as a probabilistic event depending on the survival probability given by the LR (Ps(LR)), the CT (Ps(CT)), and the NNet (Ps(NNet)) models generated from our data set. Further, the Ps(LR), Ps(CT), and Ps(NNet) were multiplied by their corresponding model accuracies (Ac(LR), Ac(CT), and Ac(NNet); respectively), giving the following equation for the BGM score survival probability: Ps(BGM) = (Ac(LR) × Ps(LR)) ∩ (Ac(CT) × Ps(CT)) ∩ (Ac(NNet) × Ps(NNet)). Additionally, the following constraints were applied to the Ps(BGM) to incorporate the best predictive features of the LR, CT, and NNet models:
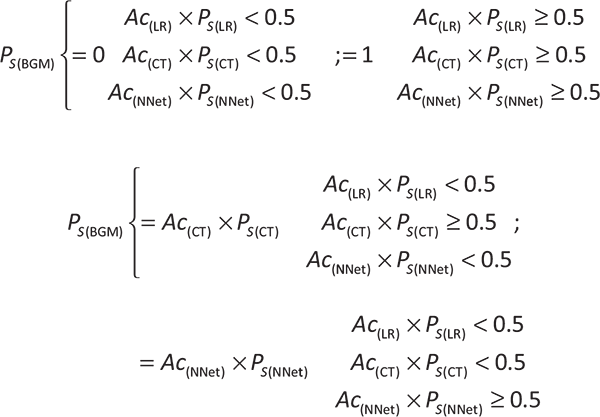
Sensitivity, specificity, positive predictive value, negative predictive value, kappa, total accuracy, and balanced accuracy ([sensitivity+specificity]/2) were calculated for each model considering only the test set (99 cases). All the statistical analyses were performed using public libraries from the Comprehensive R Archive Network (CRAN; http://CRAN.R-project.org) rooted in the open-source statistical computing environment R, version 3.6 (http://www.R-project.org/). A p-value <0.05 was considered statistically significant.
We wrote an interactive web application using the R Shiny package (14) that implements the four multivariate models that we generated in our study. This web application can be used to calculate the survival probability for a patient with COVID-19 and is freely available at the link “https://bgm-hoc.shinyapps.io/Shiny_covid_clinic/.”
Results
Five hundred subjects with a confirmed diagnosis for COVID-19 formed the study population. Overall, the median age of the patients was 64 years, 42.6% were female, and patients were discharged within a median of 10 days. The most common comorbid conditions were hypertension (44.2%), dyslipidemia (31.2%), and diabetes mellitus (18.8%). Among the patients recruited, 23.4% required ICU, and 19.4% died during follow-up. The demographic, clinical, and laboratory results of the patients corresponding to the first 48 hours after admission are summarized in Tables I and II.
We evaluated a panel of 12 biochemical, 5 hematological, and 3 coagulation biomarkers for each patient. As shown in Figure 1, we detected the presence of high significant correlations in AST-ALT (r = 0.9, p < 0.001) and WBC-NEU (r = 0.8, p < 0.001). To avoid the presence of multicollinearity bias in multivariate analysis, we excluded the variables AST and NEU for future calculations. We included the rest of the biochemical, demographic, and clinical variables in the multivariate LR model that we designed considering the occurrence of death as a response variable and that we generated performing 10-fold cross-validation. Among all the explanatory variables included initially in the model (variables shown in Tabs. I and II) only age, CREA, DD, CRP, PLT, and TNI were early independent predictors of mortality after admission (Tab. III), according to the model selection rule based on the Akaike information criterion (AIC). This multivariate model yielded a sensitivity of 47.3%, a specificity of 98.7%, a kappa of 0.56, and a balanced accuracy of 0.73 for identifying patients with a high risk of mortality. We obtained these performance characteristics using a validation set of 99 cases that were not used for training the model. Despite the high negative predictive value of the LR model (0.9), we observed a low sensitivity that suggests that the model was sensitive to class imbalance.
Total
n = 500 |
Nonsurvivors
n = 97 (19.4%) |
Survivors
n = 403 (80.6%) |
p-value | |
---|---|---|---|---|
Female, n (%) | 213 (42.6%) | 42 (43.3%) | 171 (42.4%) | 0.96752 |
Age, median (IQR) | 64 (54-76) | 80 (72-86) | 61 (50-72) | 6.50e-25 |
Active smoker, n (%) | 25 (5.0%) | 6 (6.2%) | 19 (4.7%) | 0.73589 |
Active alcohol consumer, n (%) | 15 (3.0%) | 4 (4.1%) | 11 (2.7%) | 0.69568 |
Asthma, n (%) | 25 (5.0%) | 5 (5.2%) | 20 (5.0%) | 1.00000 |
COPD, n (%) | 26 (5.2%) | 8 (8.2%) | 18 (4.5%) | 0.21092 |
Diabetes, n (%) | 94 (18.8%) | 30 (30.9%) | 64 (15.9%) | 0.00111 |
Dyslipidemia, n (%) | 156 (31.2%) | 41 (42.3%) | 115 (28.5%) | 0.01247 |
Obesity, n (%) | 33 (6.6%) | 4 (4.1%) | 29 (7.2%) | 0.38628 |
Hypertension, n (%) | 221 (44.2%) | 64 (66.0%) | 157 (39.0%) | 2.63e-06 |
Atrial fibrillation, n (%) | 37 (7.4%) | 10 (10.3%) | 27 (6.7%) | 0.31576 |
Heart failure, n (%) | 23 (4.6%) | 11 (11.3%) | 12 (3.0%) | 0.00112 |
Ischemic heart disease, n (%) | 31 (6.2%) | 14 (14.4%) | 17 (4.2%) | 0.00045 |
ICU admission, n (%) | 117 (23.4%) | 30 (30.9%) | 87 (21.6%) | 0.06921 |
Hospitalization days, median (IQR) | 10 (6-18) | 6 (3-11) | 12 (7-20) | 9.86e-09 |
COPD = chronic obstructive pulmonary disease; ICU = intensive care unit; IQR = interquartile range.
Total (n = 500) | Nonsurvivors (n = 97) | Survivors (n = 403) | p-value | Normal range | ||||
---|---|---|---|---|---|---|---|---|
n | Median (IQR) | n | Median (IQR) | n | Median (IQR) | |||
ALP, U/L | 491 (98.2%) | 68 (55-90) | 94 (96.9%) | 76 (58-108) | 397 (98.5%) | 68 (45-86) | 0.00342 | 46-116 |
ALT, U/L | 492 (98.4%) | 29 (19-50) | 95 (97.9%) | 25 (18-48) | 397 (98.5%) | 29 (19-51) | 0.30541 | 5-40 |
AST, U/L | 487 (97.4%) | 38 (27-60) | 93 (95.9%) | 49 (31-73) | 394 (97.8%) | 37 (26-56) | 0.00974 | 5-40 |
TBIL, mg/dL | 490 (98.0%) | 0.5 (0.4-0.7) | 93 (95.9%) | 0.6 (0.4-0.9) | 397 (98.5%) | 0.5 (0.4-0.7) | 0.01930 | 0.2-1.2 |
CREA, mg/dL | 500 (100%) | 0.89 (0.71-1.1) | 97 (100%) | 1.11 (0.87-1.83) | 403 (100%) | 0.86 (0.69-1.04) | 4.60e-10 | 0.3-1.3 |
FER, ng/mL | 383 (76.6%) | 602 (266-1278) | 71 (73.2%) | 914 (376-1533) | 312 (77.4%) | 559 (240-1190) | 0.00221 | 15-200 |
GGT, U/L | 491 (98.2%) | 39 (25-78) | 94 (96.9%) | 39 (27-92) | 397 (98.5%) | 40 (24-76) | 0.43835 | 5-40 |
GLU, mg/dL | 500 (100%) | 107 (96-130) | 97 (100%) | 125 (104-160) | 403 (100%) | 105 (95-123) | 9.95e-07 | 65-110 |
LDH, U/L | 477 (95.4%) | 316 (244-418) | 87 (89.7%) | 432 (276-583) | 390 (96.8%) | 301 (240-395) | 6.99e-07 | <234 |
CRP, mg/dL | 499 (99.8%) | 7.3 (3.4-15.1) | 97 (100%) | 14.3 (7.9-22.8) | 402 (99.8%) | 6.3 (2.8-11.9) | 1.51e-12 | <1 |
PCT, ng/mL | 416 (83.2%) | 0.11 (0.05-0.25) | 77 (79.4%) | 0.37 (0.17-1.05) | 339 (84.1%) | 0.09 (0.04-0.18) | 2.40e-19 | <0.5 |
TNI, ng/L | 410 (82.0%) | 8.5 (3.9-22.8) | 77 (79.4%) | 45.0 (20.1-112.1) | 333 (82.6%) | 6.8 (3.2-14.9) | 1.05e-21 | <45.2 |
HB, g/L | 500 (100%) | 137 (126-147) | 97 (100%) | 130 (114-143) | 403 (100%) | 139 (128-148) | 0.00015 | 120-170 |
PLT, ×109/L | 500 (100%) | 180 (137-227) | 97 (100%) | 166 (112-220) | 403 (100%) | 182 (146-231) | 0.00130 | 130-400 |
WBC, ×109/L | 500 (100%) | 6.0 (4.5-7.7) | 97 (100%) | 7.2 (5.4-9.8) | 403 (100%) | 5.8 (4.4-7.3) | 1.89e-05 | 4-11 |
LYMPH, ×109/L | 500 (100%) | 0.8 (0.6-1.1) | 97 (100%) | 0.6 (0.4-0.9) | 403 (100%) | 0.9 (0.6-1.2) | 5.10e-11 | 0.9-4.5 |
NEU, ×109/L | 500 (100%) | 4.6 (3.2-6.3) | 97 (100%) | 5.7 (4.5-8.3) | 403 (100%) | 4.2 (3.1-5.7) | 5.17e-08 | 2-7 |
DD, ng/mL | 450 (90.0%) | 700 (400-1300) | 79 (81.4%) | 1500 (800-4350) | 371 (92.1%) | 600 (400-1000) | 1.96e-11 | <500 |
PT, sec | 273 (54.6%) | 12.8 (12.1-13.6) | 57 (58.8%) | 13.1 (12.3-14.3) | 216 (53.6%) | 12.8 (12.1-13.5) | 0.02284 | 9.9-13.7 |
PTT, sec | 225 (45.0%) | 29.7 (27.4-31.8) | 55 (56.7%) | 29.1 (26.7-31.3) | 170 (42.2%) | 30.0 (27.9-32.0) | 0.11795 | 23.5-32.5 |
ALP = alkaline phosphatase; ALT = alanine aminotransferase; AST = aspartate aminotransferase; CREA = creatinine; CRP = C-reactive protein; DD = D-dimer; FER = ferritin; GLU = glucose; GGT = gamma-glutamyl transferase; HB = hemoglobin; LDH = lactate dehydrogenase; LYMPH = lymphocyte count; NEU = neutrophil count; PCT = procalcitonin; PLT = platelet count; PT = prothrombin time; PTT = partial thromboplastin time; TBIL = total bilirubin; TNI = troponin I; WBC = white blood cell count.
To improve our classification performance without downsampling, we next generated two additional multivariate models based on a different paradigm of categorization, CTs with the CART algorithm (the implementation in R is called rpart) and NNets. Considering all the variables from Tables I and II, the CART CT yielded TNI (cutoff value of 44 ng/L), age (cutoff value of 79 years), DD (cutoff value of 700 ng/mL), and CRP (cutoff value of 15 mg/dL) as the most potent early predictors for stratifying patients with a high vs. low risk of mortality (Fig. 2). These cutoff values were similar to their reference intervals implemented for clinical diagnosis (TNI < 45.2 ng/L, DD < 500 ng/mL, and CRP < 1 mg/dL). This model outperformed the LR model with a sensitivity of 68.4%, a specificity of 92.5%, a kappa of 0.61, and a balanced accuracy of 0.80 for identifying patients with a high risk of mortality in our validation set. Despite this performance comparison, one common characteristic in these models was that both yielded clinical laboratory measurements as the most powerful predictors of mortality in patients with COVID-19.
In general, the outputs of LR and CT are intuitive and easy to implement as predictive algorithms in the clinical setting. However, NNets are black boxes regarding the contribution of the explanatory variables to the output of the response variable. Therefore, we have to limit the selection of variables to generate manageable NNet models applicable to most clinical settings regardless of their limitations in their laboratory tests portfolio. In this context, and with the intention intending of improving our LR model, we generated an artificial NNet including only the variables that remained as early independent predictors of mortality in the LR model: age, CREA, DD, CRP, PLT, and TNI. Supplemental figure 1 shows the optimal architecture of the neural model that was obtained after 10-fold cross-validation. The NNet model yielded a sensitivity of 66.7%, a specificity of 92.3%, a kappa of 0.54, and a balanced accuracy of 0.79 for identifying patients with a high risk of mortality in our validation set. This performance was comparable to that achieved by our previous CT algorithm.
Odd ratio | Std. error | Z-statistic | p-value | |
---|---|---|---|---|
Age | 2.012 | 1.281 | 5.665 | 1.47e-08 |
CREA | 2.573 | 1.012 | 2.465 | 0.01370 |
DD | 2.086 | 1.000 | 2.493 | 0.01266 |
CRP | 2.012 | 1.828 | 4.942 | 7.71e-07 |
PLT | 0.697 | 1.079 | –3.025 | 0.00249 |
TNI | 1.210 | 1.000 | 2.051 | 0.04031 |
CREA = creatinine; CRP = C-reactive protein; DD = D-dimer; PLT = platelet count; TNI = troponin I.
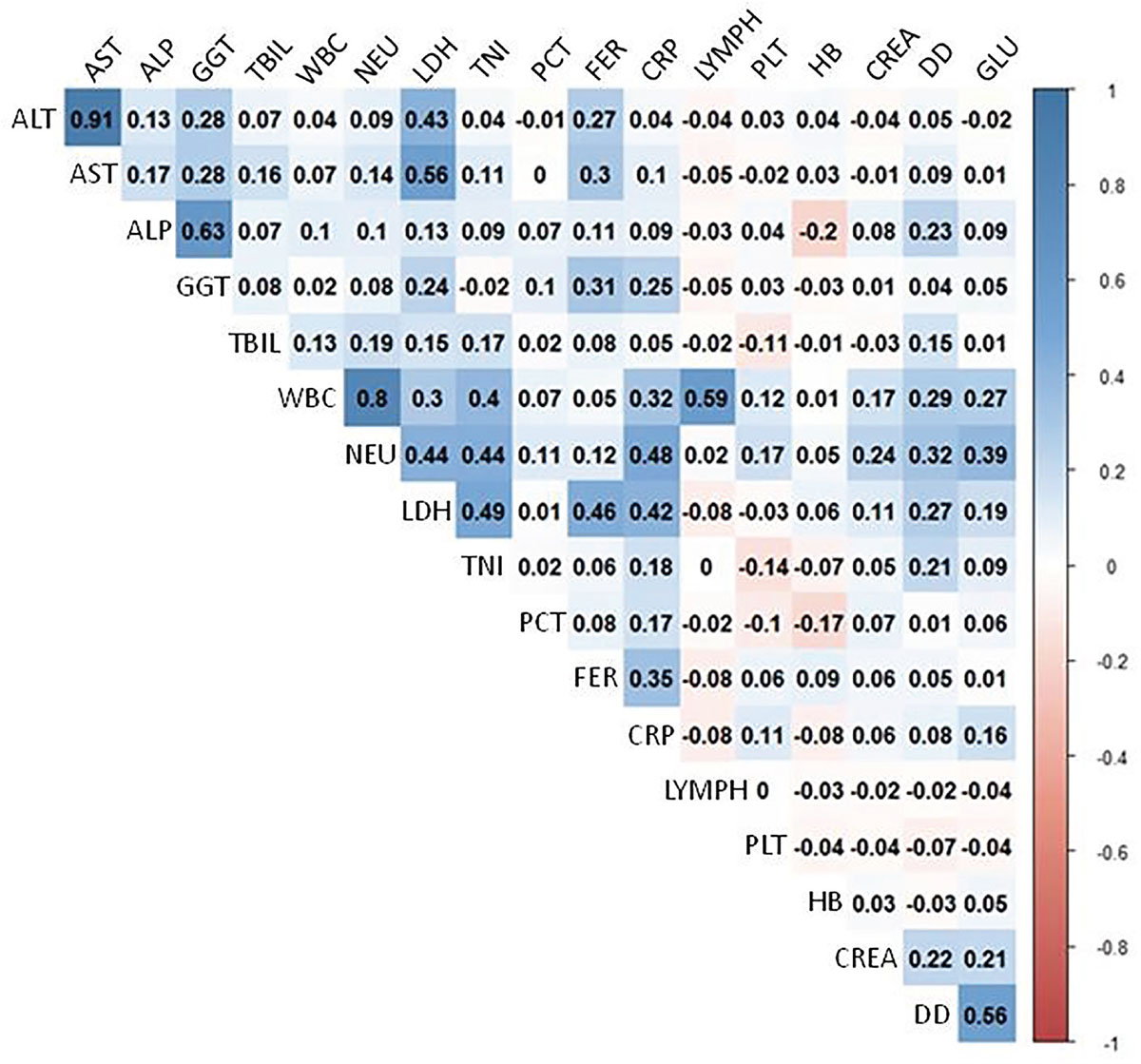
Fig. 1 - Correlation plot. The plot shows the correlation between all the clinical laboratory results obtained within the first 48 hours after patient admission. The bar on the right depicts the equivalence between the color code, and the value of the correlation coefficients shown for each pair of laboratory parameters.
ALP = alkaline phosphatase; ALT = alanine aminotransferase; AST = aspartate aminotransferase; CREA = creatinine; CRP = C-reactive protein; DD = D-dimer; FER = ferritin; GLU = glucose; GGT = gamma-glutamyl transferase; HB = hemoglobin; LDH = lactate dehydrogenase; LYMPH = lymphocyte count; NEU = neutrophil count; PCT = procalcitonin; PLT = platelet count; TBIL = total bilirubin; TNI = troponin I; WBC = white blood cell count.
The three algorithms we generated can be divided into two groups considering their sensitivity and specificity. The model with the highest specificity was LR, while the CT and NNet models presented lower specificity but a higher sensitivity. These differences in predictive accuracy led to our developing a new hybrid model in combination with the LR, CT, and NNet models to incorporate the best predictive features of each. As described in the Material and Methods, our model, called the BGM score, calculates a survival probability for patients with COVID-19 by multiplying the survival probabilities of the three previous models corrected by their accuracies. We assessed the BGM score performance in terms of prediction accuracy over the validation set, yielding a sensitivity of 73.7%, a specificity of 96.5%, a kappa of 0.74, and a balanced accuracy of 0.85 for the prediction of COVID-19 patients who died. Figure 3 shows the statistical comparison of the accuracies of the four models, where it can be seen that the BGM score model significantly outperformed all the other models. Our hybrid model corroborates the prognostic value of the clinical laboratory tests for patients with COVID-19.
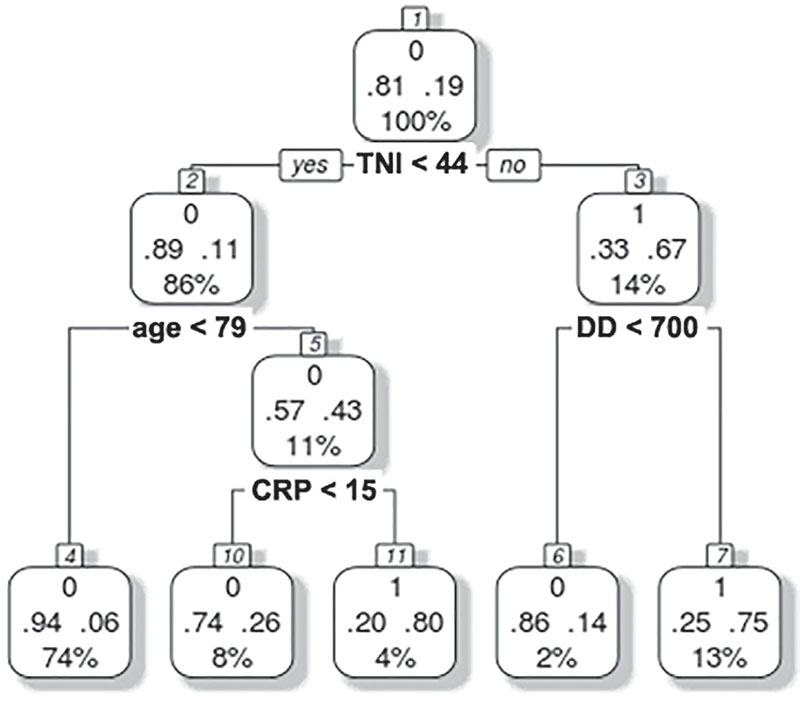
Fig. 2 - Multivariate classification tree analysis. Troponin I (TNI), age, D-dimer (DD), and C-reactive protein (CRP) were the most powerful predictors. The number 0 represents survival and 1 represents death. For each square (leaves), survival and death probabilities are represented with decimal numbers at the left and the right side, respectively, and the percentage represents the number of cases that is split between the leaves of tree partitions.
Units: TNI, ng/mL; age, years; DD, ng/mL; CRP, mg/dL.
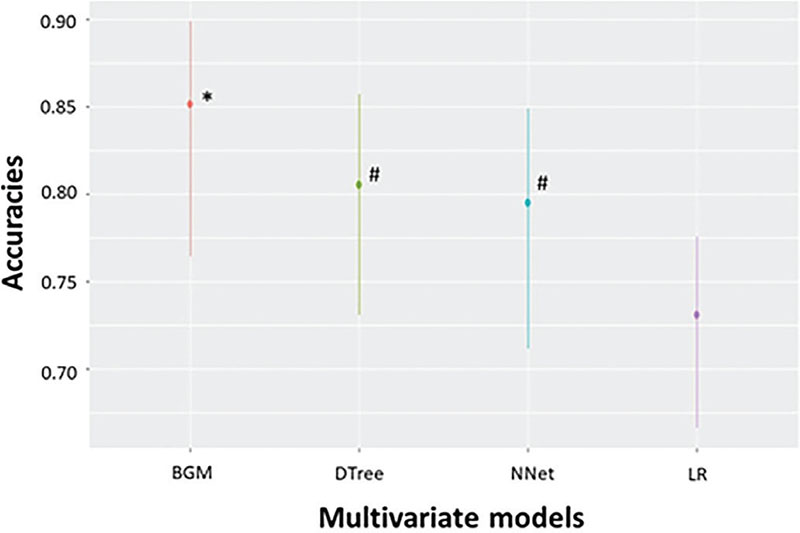
A web application with the implementation of the BGM score model is available at “https://bgm-hoc.shinyapps.io/Shiny_covid_clinic/”.
Discussion
This retrospective study identified risk factors for death in hospitalized patients with COVID-19. Older age, lower LYMPH and PLT in addition to higher CREA, DD, CRP, and TNI were independent risk factors for death among patients. Taking this into account, we developed a predictive algorithm for mortality (BGM score) considering standard laboratory tests usually present in most central clinical laboratories.
Concerning biochemical, hematological, and coagulation parameters, our findings are in consonance with those previously described. For instance, a recent published work by Sisó-Almirall et al (15) revealed that LDH, DD, and CRP were the most important laboratory parameters significantly associated with adverse outcomes, evaluated as death or ICU admission.
Alterations in coagulation parameters, in particular high DD level and low PLT, have been linked with severe COVID-19 patients (16,17). These disorders reflect the hypercoagulable state present in poor prognosis, which could promote microthrombosis in the lungs, as well as in other organs (18).
Elevated TNI levels are frequent in patients with COVID-19 and have been significantly associated with fatal outcomes. Several mechanisms may explain this phenomenon: viral myocarditis, cytokine-driven myocardial damage, microangiopathy, and unmasked coronary artery disease. SARS-CoV-2 uses angiotensin-converting enzyme 2 (ACE2) as its entry receptor and subsequently downregulates ACE2 expression. This mechanism may complicate the clinical course mediated through inflammatory response, endothelial dysfunction, and microvascular damage (19).
Early monitoring of immunological biomarkers is an important basis to guide treatment strategies in COVID-19. In this study, CRP was the only immunological biomarker assessed significantly related to mortality. Recently, a meta-analysis including 16 independent studies highlighted the importance of CRP as a possible biomarker for mortality due to COVID-19 infection (20). Furthermore, the study by Wang (21) showed that CRP levels were positively correlated with lung lesion and disease severity in the early stage of COVID-19.
Lymphopenia is a common feature in patients with COVID-19. Significant decreases in T-cell counts have been observed in patients with severe disease (22). Up to now, the underlying mechanisms leading to the observed lymphopenia are little known and better understanding will provide insight into better management of such patients (23).
A high serum CREA level is a frequently observed complication in nonsurvivor inpatients (24). It has been described that around 20% of patients admitted to an ICU require renal replacement therapy 15 days after illness onset (25).
Previous studies reported comorbidities to be one of the most important risk factors associated with increased disease severity (6,7,26). Likewise, our study reported a significant association between mortality and some of the collected comorbidities, including diabetes, dyslipidemia, hypertension, heart failure, and ischemic heart disease. Despite these differences found between survivors and nonsurvivors, none of our prediction models did include any comorbidity since the clinical laboratory measurements were stronger predictors of mortality in patients with COVID-19.
Age was the only nonlaboratory-related variable associated with symptom aggravation in our study. This is in accordance with previous publications reporting age to be the most important predictor of death in patients with COVID-19 (27). Immunosenescence is defined as the declined ability of elderly patients to react properly upon infection, to initiate and maintain an adequate protective immune response, and to develop immunological memory (28). Thus, the severity of viral infections (e.g., influenza, respiratory syncytial virus) is notably increased among older adults compared to younger individuals, and more acute and long-term sequelae often develop as a result (29,30).
Other studies have been published using machine learning models to predict the mortality in COVID-19 patients, and some of them were included in systematic reviews and meta-analysis (31). Our study presents some common points with these publications since the predictors used in the BGM score were also identified to be relevant predictors of mortality in other models, supporting their significant association with adverse patient outcomes. One of our study’s strengths is that we have used a relatively large sample size compared with the research items cited in the meta-analysis, including a substantial number of nonsurvivors. Also, our final algorithm, the BGM score, only includes a small number of simple laboratory measurements, which makes our model easy to implement in the routine clinical practice. It’s noteworthy that all the variables of our study were collected in the first 48 hours of admission. Hence, our model can provide an early detection of patients at high risk of death, favoring early interventions.
Our study has several limitations. First, it was a retrospective single-center study, which may lead to biased results. Second, we recruited only patients with moderate or severe-stage disease and not asymptomatic or mild-stage disease. Therefore, prospective multicenter studies including patients with various stages of disease are warranted to confirm the reliability of the BGM score model.
The effects the pandemic is causing on medical resources worldwide highlight the need to develop early predictor models capable of detecting which patients can be managed safely at district hospital or can benefit from domiciliary hospitalization programs and which ones will need intensive care. Therefore, identifying risk factors at presentation that predict the likelihood of disease progression will be useful to: (1) increase the therapeutic effect in patients with a risk of higher disease progression and (2) reduce the mean hospitalization time in patients not at risk.
To conclude, we have developed an easy-to-use model comprising biochemical, hematological, and coagulation parameters presented in most clinical laboratories able to predict the survival probability of a patient with COVID-19 with high accuracy. This web tool may support clinicians in managing this infectious disease and providing focused interventions to patients with COVID-19 at a higher risk of death.
Disclosures
Financial support: This work was supported by grants to MM-R (PID2019-105502RB-100) and to WJ (RTI2018-094734-B-C21) from Dirección General de Investigación Científica y Técnica and Agència de Gestió d’Ajuts Universitaris i de Recerca (SGR 2017/2019). The work was cofinanced by FEDER of European Union. The Centro de Investigación Biomédica en Red de Enfermedades Hepáticas y Digestivas (CIBERehd) is funded by Instituto de Salud Carlos III.
Conflict of interest: The authors have no financial relationships to disclose relevant to this study.
References
- 1. The Lancet. Emerging understandings of 2019-nCoV. Lancet. 2020;395(10221):311. CrossRef PubMed
- 2. Zhu N, Zhang D, Wang W, et al; China Novel Coronavirus Investigating and Research Team. A novel coronavirus from patients with pneumonia in China, 2019. N Engl J Med. 2020;382(8):727-733. CrossRef PubMed
- 3. World Health Organization. Report of the WHO-China Joint Mission on Coronavirus Disease 2019 (COVID-19). Online (Accessed September 2020).
- 4. European Centre for Disease Prevention and Control. Novel coronavirus disease 2019 (COVID-19) pandemic: increased transmission in the EU/EEA and the UK – sixth update. Online (Accessed September 2020).
- 5. Richardson S, Hirsch JS, Narasimhan M, et al; the Northwell COVID-19 Research Consortium. Presenting Characteristics, Comorbidities, and Outcomes Among 5700 Patients Hospitalized With COVID-19 in the New York City Area. JAMA. 2020;323(20):2052-2059. CrossRef PubMed
- 6. Chen N, Zhou M, Dong X, et al. Epidemiological and clinical characteristics of 99 cases of 2019 novel coronavirus pneumonia in Wuhan, China: a descriptive study. Lancet. 2020;395(10223):507-513. CrossRef PubMed
- 7. Wang D, Hu B, Hu C, et al. Clinical Characteristics of 138 Hospitalized Patients With 2019 Novel Coronavirus-Infected Pneumonia in Wuhan, China. JAMA. 2020;323(11):1061-1069. CrossRef PubMed
- 8. Hou H, Zhang B, Huang H, et al. Using IL-2R/lymphocytes for predicting the clinical progression of patients with COVID-19. Clin Exp Immunol. 2020;201(1):76-84. CrossRef PubMed
- 9. Shen B, Yi X, Sun Y, et al. Proteomic and Metabolomic Characterization of COVID-19 Patient Sera. Cell. 2020;182(1):59-72.e15. CrossRef PubMed
- 10. Messner CB, Demichev V, Wendisch D, et al. Ultra-High-Throughput Clinical Proteomics Reveals Classifiers of COVID-19 Infection. Cell Syst. 2020;11(1):11-24.e4. CrossRef PubMed
- 11. Kuhn M. caret: Classification and Regression Training. R package version 6.0-86; 2020. Online
- 12. Therneau T, Atkinson B, Ripley B, Ripley MB. rpart: Recursive Partitioning and Regression Trees. R Packag version 41-10; 2019. Online
- 13. Venables WN, Ripley BD. Modern Applied Statistics With S. 4th ed. New York: Springer; 2002. CrossRef
- 14. Chang W, Cheng J, Allaire J, Xie Y, McPherson J. shiny: Web Application Framework for R. R package version 1.5.0;2020. Online
- 15. Sisó-Almirall A, Kostov B, Mas-Heredia M, et al. Prognostic factors in Spanish COVID-19 patients: A case series from Barcelona. PLoS One. 2020;15(8):e0237960. CrossRef PubMed
- 16. Tang N, Li D, Wang X, Sun Z. Abnormal coagulation parameters are associated with poor prognosis in patients with novel coronavirus pneumonia. J Thromb Haemost. 2020;18(4):844-847. CrossRef PubMed
- 17. Lippi G, Plebani M, Henry BM. Thrombocytopenia is associated with severe coronavirus disease 2019 (COVID-19) infections: A meta-analysis. Clin Chim Acta. 2020;506:145-148. CrossRef PubMed
- 18. Salamanna F, Maglio M, Landini MP, Fini M. Platelet functions and activities as potential hematologic parameters related to Coronavirus Disease 2019 (Covid-19). Platelets. 2020;31(5):627-632. CrossRef PubMed
- 19. Tersalvi G, Vicenzi M, Calabretta D, Biasco L, Pedrazzini G, Winterton D. Elevated Troponin in Patients With Coronavirus Disease 2019: possible Mechanisms. J Card Fail. 2020; 26(6):470-475. CrossRef PubMed
- 20. Sahu BR, Kampa RK, Padhi A, Panda AK. C-reactive protein: A promising biomarker for poor prognosis in COVID-19 infection. Clin Chim Acta. 2020;509:91-94. CrossRef PubMed
- 21. Wang L. C-reactive protein levels in the early stage of COVID-19. Med Mal Infect. 2020;50(4):332-334. CrossRef PubMed
- 22. Liu Z, Long W, Tu M, et al. Lymphocyte subset (CD4+, CD8+) counts reflect the severity of infection and predict the clinical outcomes in patients with COVID-19. J Infect. 2020;81(2):318-356. CrossRef PubMed
- 23. Tavakolpour S, Rakhshandehroo T, Wei EX, Rashidian M. Lymphopenia during the COVID-19 infection: what it shows and what can be learned. Immunol Lett. 2020;225:31-32. CrossRef PubMed
- 24. Li Z, Wu M, Yao J, et al. Caution on Kidney Dysfunctions of COVID-19 Patients. Preprint Online (2020).
- 25. Zhou F, Yu T, Du R, et al. Clinical course and risk factors for mortality of adult inpatients with COVID-19 in Wuhan, China: a retrospective cohort study. Lancet. 2020;395(10229):1054-1062. CrossRef PubMed
- 26. Chang MC, Park YK, Kim BO, Park D. Risk factors for disease progression in COVID-19 patients. BMC Infect Dis. 2020;20(1):445. CrossRef PubMed
- 27. Porcheddu R, Serra C, Kelvin D, Kelvin N, Rubino S. Similarity in Case Fatality Rates (CFR) of COVID-19/SARS-COV-2 in Italy and China. J Infect Dev Ctries. 2020;14(2):125-128. CrossRef PubMed
- 28. Reber AJ, Chirkova T, Kim JH, et al. Immunosenescence and challenges of vaccination against influenza in the aging population. Aging Dis. 2012;3(1):68-90. PubMed
- 29. Gordon A, Reingold A. The Burden of Influenza: a Complex Problem. Curr Epidemiol Rep. 2018;5(1):1-9. CrossRef PubMed
- 30. Gozalo PL, Pop-Vicas A, Feng Z, Gravenstein S, Mor V. Effect of influenza on functional decline. J Am Geriatr Soc. 2012;60(7):1260-1267. CrossRef PubMed
- 31. Wynants L, Van Calster B, Collins GS, et al. Prediction models for diagnosis and prognosis of covid-19 infection: systematic review and critical appraisal. BMJ. 2020;369:m1328. CrossRef PubMed