![]() |
Drug Target Insights 2023; 17: 58-69 ISSN 1177-3928 | DOI: 10.33393/dti.2023.2595 ORIGINAL RESEARCH ARTICLE |
![]() |
Network analysis for identifying potential anti-virulence targets from whole transcriptome of Pseudomonas aeruginosa and Staphylococcus aureus exposed to certain anti-pathogenic polyherbal formulations
ABSTRACT
Introduction: Antimicrobial resistance (AMR) is a serious global threat. Identification of novel antibacterial targets is urgently warranted to help antimicrobial drug discovery programs. This study attempted identification of potential targets in two important pathogens Pseudomonas aeruginosa and Staphylococcus aureus.
Methods: Transcriptomes of P. aeruginosa and S. aureus exposed to two different quorum-modulatory polyherbal formulations were subjected to network analysis to identify the most highly networked differentially expressed genes (hubs) as potential anti-virulence targets.
Results: Genes associated with denitrification and sulfur metabolism emerged as the most important targets in P. aeruginosa. Increased buildup of nitrite (NO2) in P. aeruginosa culture exposed to the polyherbal formulation Panchvalkal was confirmed through in vitro assay too. Generation of nitrosative stress and inducing sulfur starvation seemed to be effective anti-pathogenic strategies against this notorious gram-negative pathogen. Important targets identified in S. aureus were the transcriptional regulator sarA, immunoglobulin-binding protein Sbi, serine protease SplA, the saeR/S response regulator system, and gamma-hemolysin components hlgB and hlgC.
Conclusion: Further validation of the potential targets identified in this study is warranted through appropriate in vitro and in vivo assays in model hosts. Such validated targets can prove vital to many antibacterial drug discovery programs globally.
Keywords: AMR (antimicrobial resistance), Anti-virulence, Network Analysis, Novel antibacterial targets, Polyherbal, Protein-Protein Interaction (PPI)
Received: April 29, 2023
Accepted: May 10, 2023
Published online: May 29, 2023
This article includes supplementary material
Corresponding author:
Vijay O. Kothari
Institute of Science
Nirma University
S-G Highway
Ahmedabad-382481 - India
vijay.kothari@nirmauni.ac.in
Drug Target Insights - ISSN 1177-3928 - www.aboutscience.eu/dti
© 2023 The Authors. This article is published by AboutScience and licensed under Creative Commons Attribution-NonCommercial 4.0 International (CC BY-NC 4.0).
Commercial use is not permitted and is subject to Publisher’s permissions. Full information is available at www.aboutscience.eu
Introduction
Despite wide recognition of antimicrobial resistance (AMR) as a major global health threat, the progress on discovery and development of new antibiotics in the last three to four decades clearly has fallen short from being satisfactory. For a variety of reasons, for example, lack of interest among major pharmaceutical firms, rapid emergence and spread of resistance among pathogenic bacterial populations, dearth of new validated cellular and molecular targets, the list of effective antimicrobials available for treatment of resistant infections remains short. The status of antibiotic discovery research has been reviewed thoroughly (1,2,3,4). Since most currently available antibiotics target a narrow range of bacterial traits, that is, cell envelope synthesis, protein or nucleic acid synthesis, or folic acid synthesis, a truly new class of antibiotics will be discovered only if we have a longer list of validated targets. Development of new bactericidal antibiotics is not the only way of tackling the slow pandemic of AMR infections; discovery of resistance modifiers and non-antibiotic virulence-attenuating agents can also be of great value (5,6). Hence identification of new potential targets for both bactericidal antibiotics as well as antibiotic adjuvants is useful. There is a clear need for antibiotics with previously unexploited new targets and wide target diversity in the discovery pipeline. One of the major challenges in antibacterial discovery is associated with the proper target selection, for example, the requirement of pursuing molecular targets that are not prone to rapid resistance development (7).
Various public health agencies like CDC (Centers for Disease Control and Prevention, USA), WHO (World Health Organization), and DBT (Department of Biotechnology, India) have published lists of priority pathogens against which novel antimicrobials need to be discovered urgently. Antibiotic-resistant strains of Pseudomonas aeruginosa and Staphylococcus aureus commonly appear on all such lists. As per CDC’s Vital Signs report (https://www.cdc.gov/vitalsigns/index.html) more than 33% of the bloodstream infections in patients on dialysis in the United States in 2020 were caused by S. aureus. This gram-positive human commensal has been recognized as an important opportunistic pathogen responsible for a wide range of infections (8). P. aeruginosa is the primary cause of gram-negative nosocomial infections. Its ability to adapt to a wide range of environmental niches combined with its nutritional versatility and genome plasticity, along with a multitude of intrinsic and acquired resistance mechanisms make it one of the most notorious pathogens of critical clinical importance. Efforts for finding perturbants capable of targeting the P. aeruginosa pathogenicity and antibiotic resistance are highly desired (9).
We had previously studied the anti-virulence effect of certain polyherbal formulations against S. aureus or P. aeruginosa at the whole transcriptome level of the target pathogen, wherein we gained some insight into the molecular mechanisms associated with the virulence-attenuating potential of the test formulations, which was largely independent of any growth-inhibitory effect. Pathogens exposed to the test formulations were compromised in their ability to kill the model host Caenorhabditis elegans. The current study attempted network analysis of the differentially expressed genes (DEG) of P. aeruginosa and S. aureus exposed to the anti-pathogenic polyherbal formulations Panchvalkal (10) and Herboheal (11), respectively, reported in the previous studies, with an aim to identify highly networked genes as potential anti-virulence targets. Panchvalkal is a mixture of bark extracts of five different plants – Ficus benghalensis, Ficus religiosa, Ficus racemosa, Ficus lacor, and Albizia lebbeck. Herboheal comprised of extracts of six different plants. Its full composition can be seen at: https://downloads.hindawi.com/journals/aps/2019/1739868.f1.pdf
Methods
Network analysis
We accessed the list of DEG for Panchvalkal (PentaphyteP-5®)-exposed P. aeruginosa (NCBI Bioproject ID 386078) and Herboheal-exposed S. aureus (NCBI Bioproject ID 427073). The P. aeruginosa used was a multidrug-resistant strain. Network analysis for both the studies was carried out independently, wherein only the DEG fulfilling the dual filter criteria of log fold change ≥2 and False Discovery Rate (FDR) ≤0.01 were selected for further analyses. The list of such DEG was fed into the database STRING (v. 11.5) (12) for generating the PPI (Protein-Protein Interaction) network. Then the genes were arranged in decreasing order of ‘node degree’ (a measure of connectivity with other genes or proteins), and those above a certain threshold value were subjected to ranking by cytoHubba (v. 3.9.1) (13). Since cytoHubba uses 12 different ranking methods, we considered the DEG being top-ranked by more than six different methods (i.e., 50% of the total ranking methods) for further analysis. These top-ranked shortlisted proteins were further subjected to network cluster analysis through STRING and those which were part of multiple clusters were considered ‘hubs’ which can be taken up for further validation of their targetability. Here ‘hub’ refers to a gene or protein interacting with many other genes/proteins. Hubs thus identified were further subjected to co-occurrence analysis to see whether an anti-virulence agent targeting them is likely to satisfy the criterion of selective toxicity (i.e., targeting the pathogen without harming the host). This sequence of analysis allowed us to end with a limited number of proteins which satisfied various statistical and biological significance criteria simultaneously, that is, (i) log fold change ≥2; (ii) FDR ≤0.01; (iii) relatively higher node degree; (iv) top-ranking by at least six cytoHubba methods; (v) (preferably) member of more than one local network cluster; and (vi) high probability of the target being absent from the host. A schematic presentation of the methodology employed for network analysis is presented in Figure 1.
Nitrite estimation
Nitrite estimation in P. aeruginosa culture supernatant was done through Griess assay (14). P. aeruginosa strain studied by us is a multidrug-resistant strain, which is resistant to ampicillin (10 µg), augmentin (30 µg), nitrofurantoin (300 µg), clindamycin (2 µg), chloramphenicol (30 µg), cefixime (5 µg), and vancomycin (30 µg). This bacterium was grown in Pseudomonas broth (HiMedia, Mumbai) with or without Panchvalkal (547 μg/mL; dried extract powder without any bulking agent was procured from Dr. Palep’s Medical Education and Research Foundation Pvt. Ltd., Mumbai, India, and dissolved in dimethylsulfoxide (DMSO) for assay purpose) at 35°C for 21±1 hour. Following incubation, cell density was quantified at 764 nm (15), and then the bacterial culture suspension was centrifuged at 13,600 g for 10 minutes. Resulting supernatant was mixed with Griess reagent (Sigma-Aldrich) in 1:1 ratio and incubated for 15 minutes in the dark at room temperature. Absorbance of the resulting pink color was quantified at 540 nm (Agilent Technology Cary 60 UV-Vis). These optical density (OD) values were plotted on standard curve prepared using NaNO2 to calculate the nitrite concentration. To nullify any effect of variation in cell density between control and experimental culture, nitrite unit (i.e., nitrite produced per unit of growth) was calculated by dividing the nitrite concentration values by cell density. Sodium nitroprusside (Astron chemicals, Ahmedabad) being a chemical known to be capable of generating nitrosative stress in bacteria (16,17,18) was used as a positive control. Appropriate vehicle control (i.e., bacteria grown in the presence of 0.5% v/v DMSO (Merck)), negative control (deionized water), and abiotic control (Panchvalkal-supplemented Pseudomonas broth) were included in the experiment. Griess reagent was added in all these controls in the same proportion as that in extract-exposed or not-exposed bacterial culture samples.
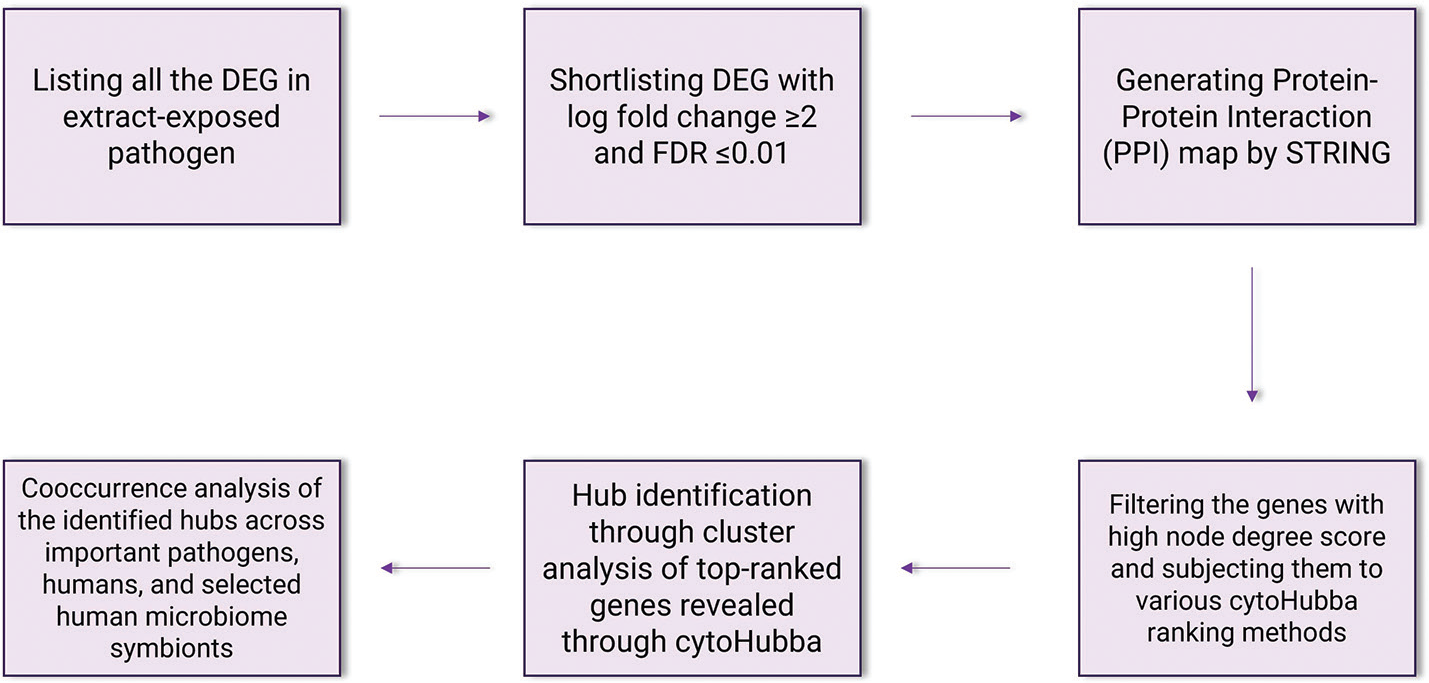
Fig. 1 - A schematic of methodology for network analysis and hub identification.
Results
Network analysis of DEG in Panchvalkal-exposed P. aeruginosa
Our original experimental study exposed P. aeruginosa to Panchvalkal at 567 μg/mL, wherein the extract-exposed pathogen could kill 90% lesser host worms than its extract-not-exposed counterpart. Whole transcriptome study revealed that approximately 14% of the P. aeruginosa genome was expressed differently under the influence of Panchvalkal. The total number of DEG satisfying the dual criteria of log fold change ≥2 and FDR ≤0.01 was 228, of which 105 were downregulated (Tab. S1) and 123 were upregulated (Tab. S4). We created PPI network for up- and downregulated genes separately (Figs. 5 and 2, respectively). PPI network for downregulated genes generated through STRING is presented in Figure 2, which shows 101 nodes connected (105 genes were fed to string, out of which 101 were shown in the PPI network) through 86 edges with an average node degree of 1.7. Since the number of edges (86) in this PPI network is 3.18-fold higher than expected (27) with a PPI enrichment p value <1.0e-16, this network can be said to possess significantly more interactions among the member proteins than what can be expected for a random set of proteins of identical sample size and degree distribution. Such an enrichment can be taken as an indication of the member proteins being at least partially biologically connected. When we arranged the 105 downregulated genes in decreasing order of node degree, 52 nodes were found to have a nonzero score (Tab. S2), and we selected top 13 genes with a node degree ≥6 for further ranking by different cytoHubba methods. Then we looked for genes which appeared among the top-10 ranked candidates by ≥6 cytoHubba methods, and 10 such shortlisted genes (Tab. S3) were further checked for interactions among themselves followed by cluster analysis (Fig. 3), which showed them to be strongly networked as the average node degree score was 8. This network possessed 40 edges as against expected (zero) for any such random set of proteins (PPI enrichment p value <1.0e-16). The PPI network generated through STRING showed these 10 important genes to be distributed among three different local network clusters. Five (norB, norC, norD, nirS, and nirQ) of the predicted hubs were part of each of the three clusters, and they have a role in denitrification (19). Of the remaining five predicted hub proteins, one more (norE) is also associated with nitrogen metabolism, and two (nosL and nosY) have a role in denitrification as well as copper homeostasis. These three proteins were members of two out of three clusters. The eight proteins (Tab. I) found to be members of minimum two clusters can be said to be potential hubs, whose downregulation can be hypothesized to attenuate P. aeruginosa virulence.
Since all the targets mentioned in Table I are known to play an important role in P. aeruginosa with respect to detoxification of reactive nitrogen species, we hypothesized that Panchvalkal-treated P. aeruginosa’s ability to detoxify reactive nitrogen species is compromised. To check this hypothesis, we quantified nitrite concentration in extract-treated P. aeruginosa culture, wherein it was found to have 31% higher nitrite concentration in supernatant as compared to control (Fig. 4). This higher accumulation of nitrite can be taken as an indication of compromised denitrification efficiency as nitrite is an intermediate of denitrification pathway (22).
PPI network for upregulated genes in Panchvalkal-exposed P. aeruginosa generated through STRING is presented in Figure 5, which shows 121 nodes connected through 70 edges with an average node degree of 1.16. Though empirically the centrality of the upregulated genes appeared to be lesser than those downregulated in Panchvalkal-exposed P. aeruginosa, since the number of edges (70) in this PPI network is 1.89-fold higher than expected (37) with a PPI enrichment p value of 1.27e-06, this network can be said to possess significantly more interactions among the member proteins than what can be expected for a random set of proteins of this much sample size and degree distribution. Such an enrichment can be taken as an indication of the member proteins being at least partially biologically connected. When we arranged the 121 upregulated genes in decreasing order of node degree, 62 nodes were found to have a nonzero score, and we selected the top 26 genes with a node degree ≥3 (Tab. S5) for further ranking by different cytoHubba methods. Then we looked for genes which appeared among top-ranked candidates by ≥6 cytoHubba methods, and 14 such genes (Tab. S6) were identified for further cluster analysis. Interaction map of these 14 important genes (Fig. 6) showed them to be networked with the average node degree score of 2.29. Number of edges possessed by this network was 16 as against expected 1 for any such random set of proteins. These 14 genes were found to be distributed among five different local network clusters. Strength score for each of these clusters was >1.5. While three of the proteins (atsB, msuE, and ssuB1) were common members of three different clusters, one gene (tauA) appeared in two clusters. All these four highly networked upregulated genes (Tab. II) are involved in sulfur metabolism in P. aeruginosa (23). Hence it may be speculated that Panchvalkal has induced sulfur starvation in P. aeruginosa, to overcome which the pathogen is forced to upregulate genes involved in sulfur transport and metabolism.
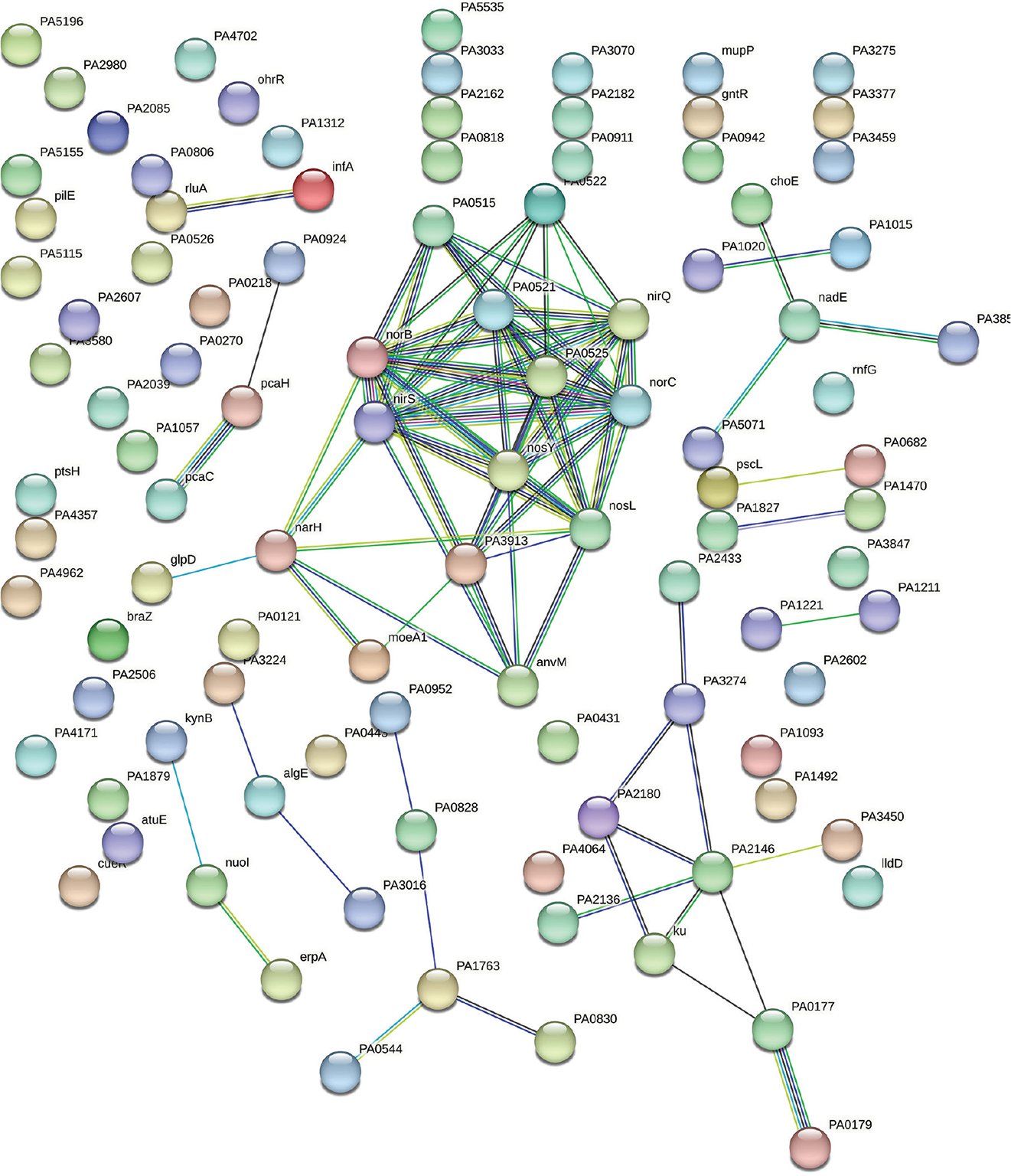
Fig. 2 - Protein-Protein Interaction (PPI) network of downregulated genes in Panchvalkal-exposed Pseudomonas aeruginosa. Edges represent protein-protein associations that are meant to be specific and meaningful, that is, proteins jointly contribute to a shared function; this does not necessarily mean they are physically binding to each other. Network nodes represent proteins. Splice isoforms or post-translational modifications are collapsed, that is, each node represents all the proteins produced by a single, protein-coding gene locus.
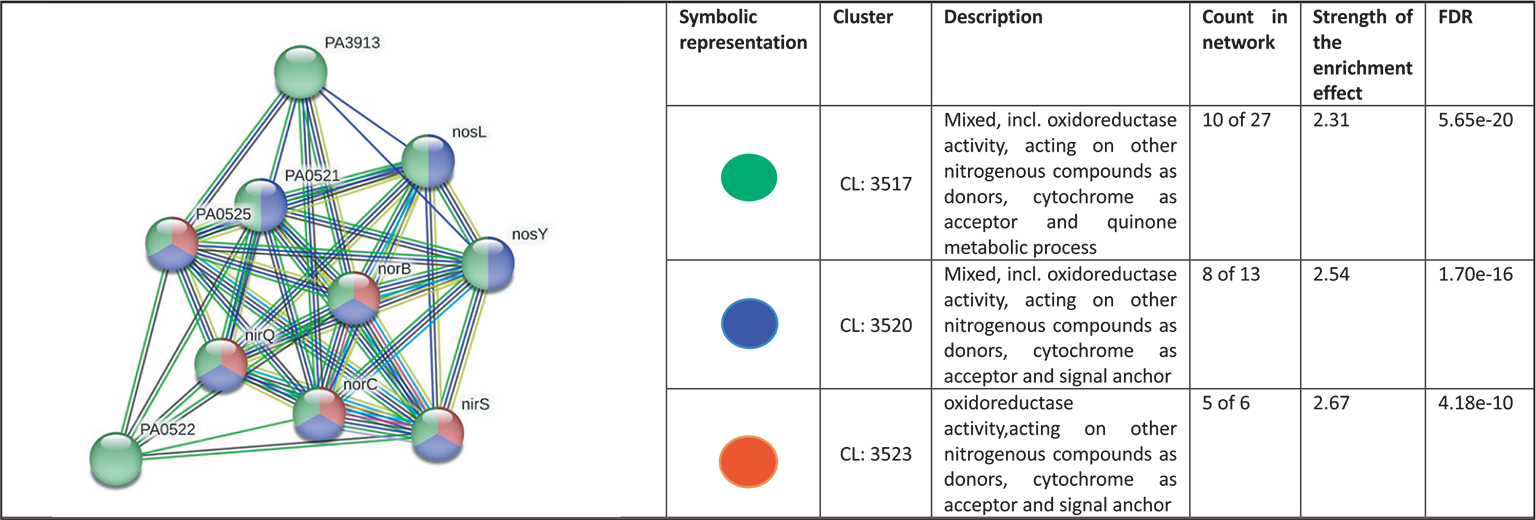
Fig. 3 - Protein-Protein Interaction (PPI) network of top-ranked genes revealed through cytoHubba among downregulated differentially expressed genes (DEG) in Panchvalkal-exposed Pseudomonas aeruginosa.
No. | Gene ID | Gene name | Functional role |
---|---|---|---|
1 | PA0520 | nirQ | Denitrification regulatory protein NirQ |
2 | PA0519 | nirS | Heme d1 biosynthesis protein, which is important for denitrification (20) |
3 | PA0524 | norB | Nitric oxide reductase subunit B |
4 | PA0523 | norC | Nitric oxide reductase subunit C |
5 | PA0525 | NorD | Nitric oxide reductase NorD protein |
6 | PA0521 | NorE | Nitric oxide reductase NorE protein |
7 | PA3395 | nosY | Nitrous oxide reductase; a Cu-processing system permease protein having role in denitrification pathway (21) |
8 | PA3396 | nosL | A lipoprotein attached to the outer membrane described as a copper-binding protein. Regulator of nos operon, NosR also associates with NosL. This protein is probably responsible for the insertion and coordination of the multicopper center within NosZ (22). |
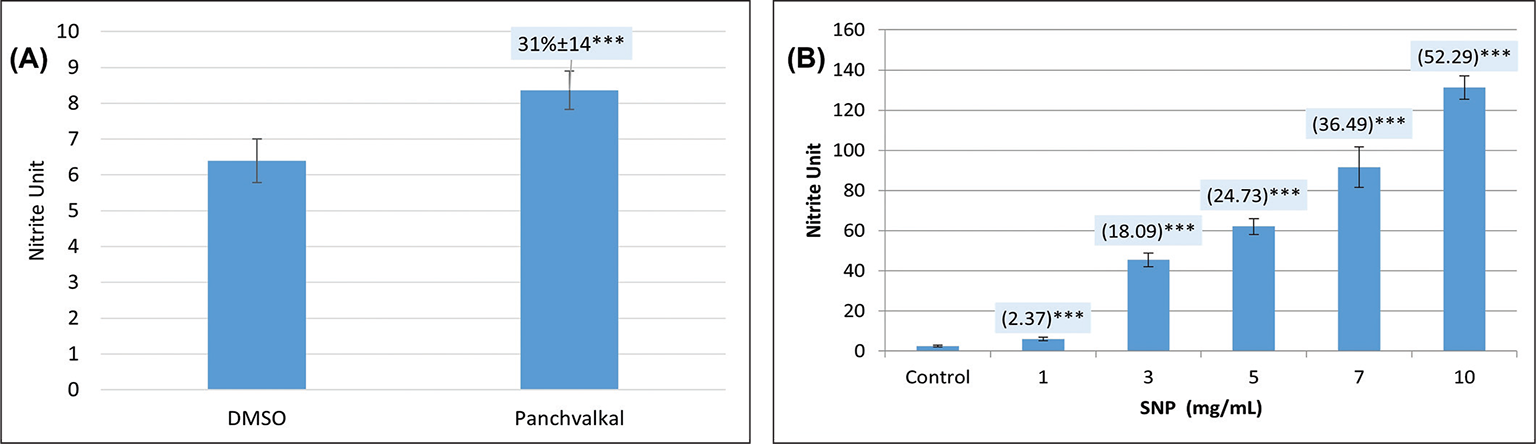
Fig. 4 - Panchvalkal-treated Pseudomonas aeruginosa culture has higher extracellular accumulation of nitrite. While nitrite concentration in vehicle control (P. aeruginosa incubated in media supplemented with 0.5% v/v dimethylsulfoxide (DMSO)) was at par to that without DMSO, Panchvalkal caused nitrite concentration in P. aeruginosa culture supernatant to rise (A). Sodium nitroprusside used as positive control caused a dose-dependent 2.37 to 52.29-fold higher nitrite buildup in P. aeruginosa culture (B). Nitrite unit (i.e., nitrite concentration:cell density ratio) was calculated to nullify any effect of cell density on nitrite production. ***p<0.001.
Network analysis of DEG in Herboheal-exposed S. aureus
Herboheal is a folk-inspired wound-healing formulation, and we had earlier demonstrated its anti-virulence potential against multiple bacterial pathogens including S. aureus. Pretreatment of S. aureus with Herboheal (0.1% v/v) could attenuate its virulence toward the surrogate host C. elegans by 55%. This concentration had a moderate growth-inhibitory effect (32%) on S. aureus, while heavily inhibiting staphyloxanthin production (79%). Whole transcriptome study revealed that approximately 17% of the S. aureus genome was expressed differently under the influence of Herboheal. The total number of DEG satisfying the dual criteria of log fold change ≥2 and FDR ≤0.01 was 113, of which 57 were upregulated and 56 were downregulated (Tab. S7). Since the number of genes amenable to mapping by STRING turned out to be only 28 of these 113, we went for a combined PPI network (Fig. 7) of all these DEG instead of preparing separate PPI map of upregulated or downregulated genes. The said PPI network had 28 nodes connected through 36 edges with an average node degree of 2.57. Since the number of edges (36) in this PPI network is threefold higher than expected (12) with a PPI enrichment p value of 1.02e-08, this network can be said to possess significantly more interactions among the member proteins than what can be expected for a random set of proteins having identical sample size and degree distribution. Such an enrichment is suggestive of the member proteins being at least partially biologically connected.
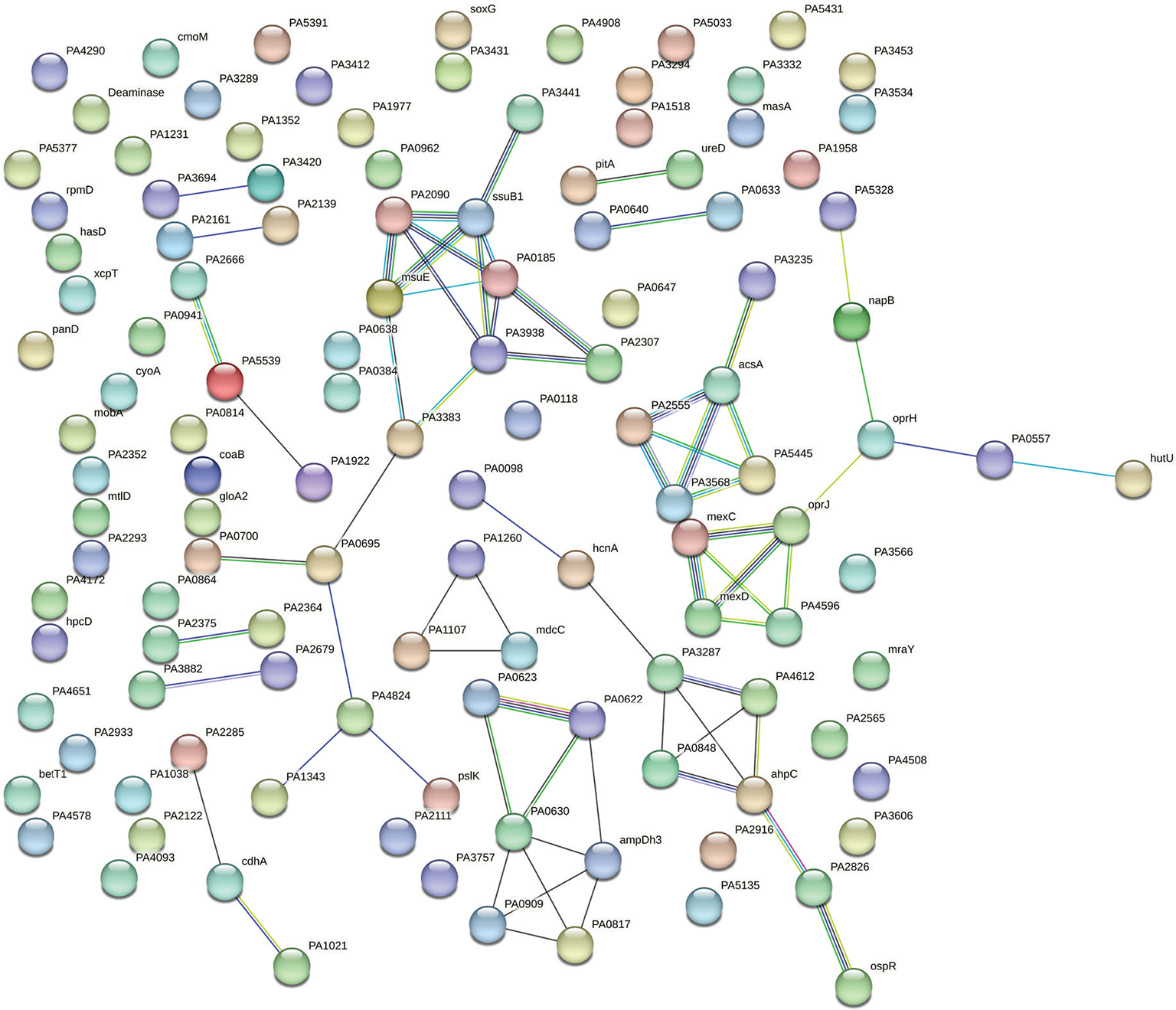
Fig. 5 - Protein-Protein Interaction (PPI) network of up-regulated genes in Panchvalkal-exposed P. aeruginosa.
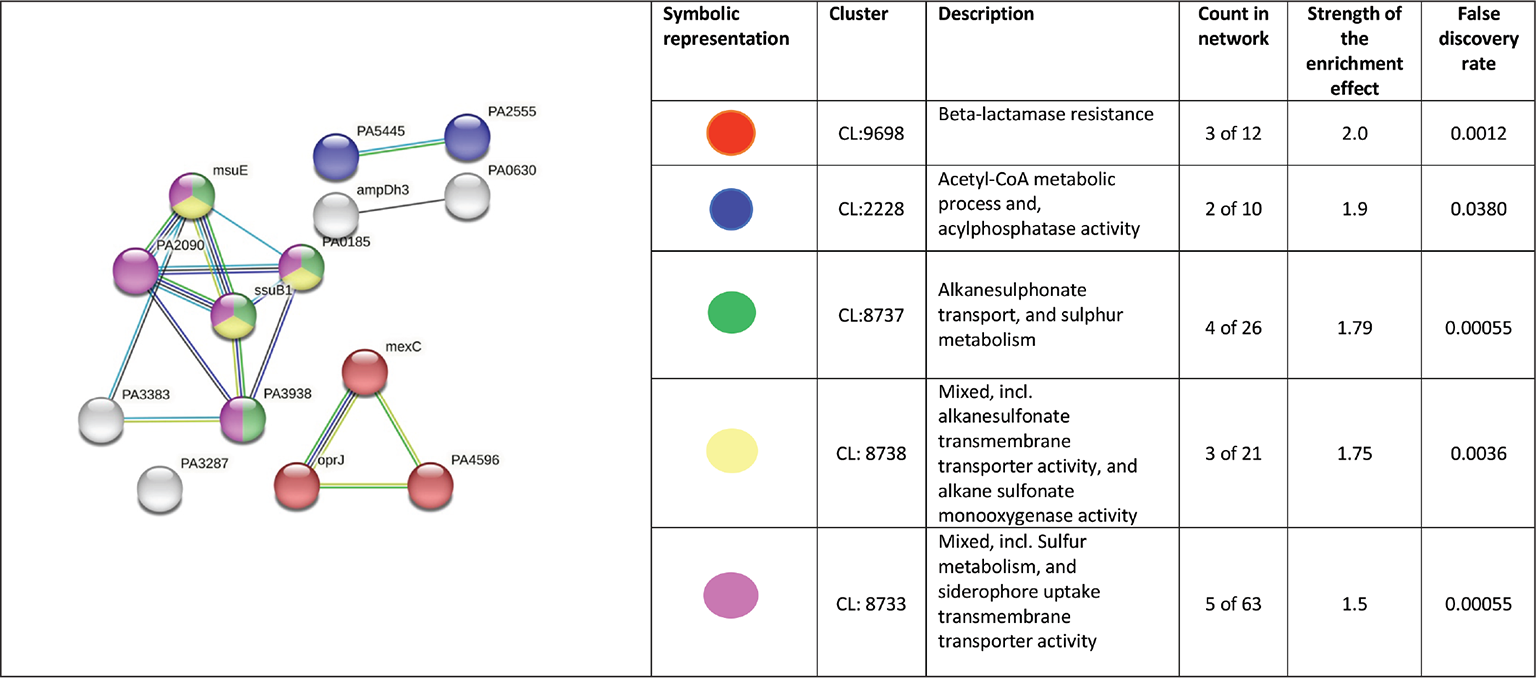
Fig. 6 - PPI network of top-ranked genes revealed through cytoHubba among up-regulated DEG in Panchvalkal-exposed P. aeruginosa.
No. | Gene ID/name | Codes for | Remarks |
---|---|---|---|
1 | PA2357/msuE (slfA) | FMN reductase | Involved in riboflavin metabolism and sulfur metabolism pathways |
2 | PA3442/ssub1 | Aliphatic sulfonates import ATP-binding protein SsuB 1 | Aliphatic sulfonates import ATP-binding protein SsuB 1; part of the ABC transporter complex SsuABC involved in aliphatic sulfonate import. Responsible for energy coupling to the transport system |
3 | PA0185/atsB | Serine-modifying enzyme (24); probable permease of ABC transporter | atsB is a member of a cys regulon in P. aeruginosa, which constitutes a general sulfate ester transport system (25) |
4 | PA3938/tauA | TauA (sulfonate transport system ATP-binding protein) | This probable periplasmic taurine-binding protein precursor is part of tau operon involved in sulfur metabolism |
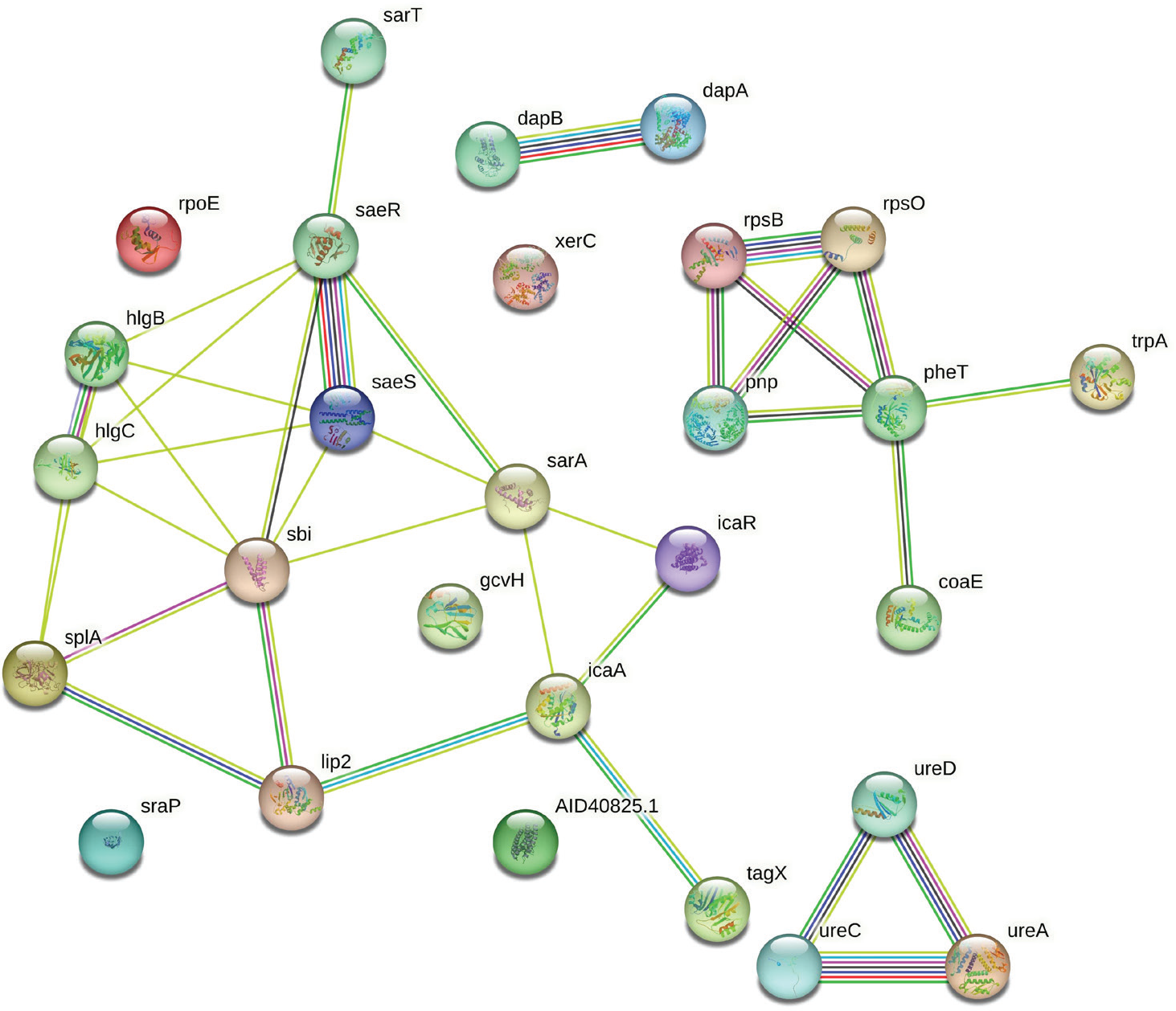
Fig. 7 - Protein-Protein Interaction (PPI) network of upregulated and downregulated genes in Herboheal-exposed Staphylococcus aureus.
When we arranged all the 28 nodes in decreasing order of node degree, 23 nodes were found to have a nonzero score, and we selected the top 13 genes with a node degree ≥3 (Tab. S8) for further ranking by different cytoHubba methods. Then we looked for genes which appeared among top-ranked candidates by ≥6 cytoHubba methods. Of such 12 genes, 8 (Tab. S9) which were ranked among top 10 by ≥11 cytoHubba methods were taken for further cluster analysis. Interaction map of these eight important genes (Fig. 8) showed them to be networked with the average node degree score of 4. Number of edges possessed by this network was 16 as against expected 1 for any such random set of proteins. These eight genes were found to be distributed among three different local network clusters. Strength score for each of these clusters was >1.46. While three of the proteins (sarA, sbi, and splA) were common members of two different clusters, four proteins were part of any one cluster, while pnp was not shown to be connected to the remaining seven genes. Since in case of S. aureus, we analyzed up- and downregulated genes together, instead of considering only the multi-cluster proteins as hubs, we took all of those which appeared to be part of PPI network as shown in Figure 7. Functions of these seven potential hubs are listed in Table III.
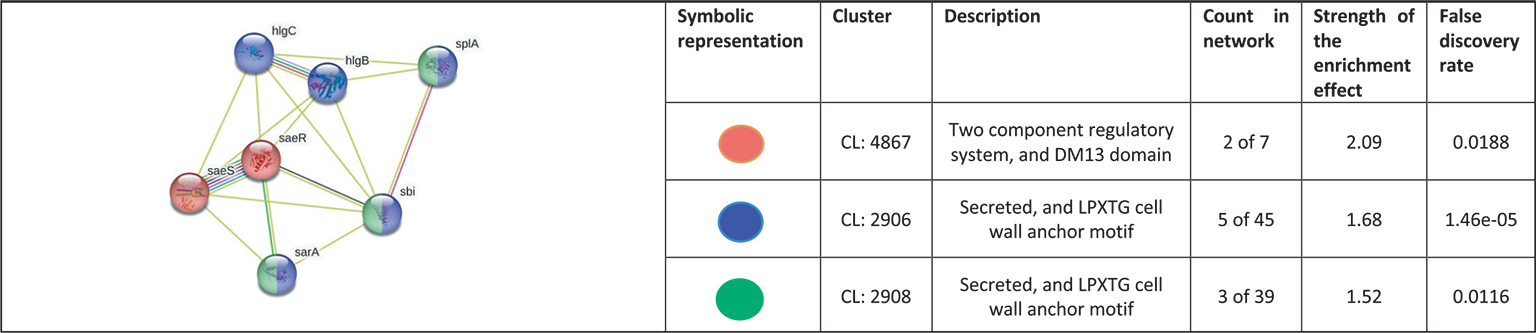
Fig. 8 - Protein-Protein Interaction (PPI) network of top-ranked genes revealed through cytoHubba among differentially expressed genes (DEG) in Herboheal-exposed Staphylococcus aureus.
No. | Gene ID | Gene name | Codes for | Function |
---|---|---|---|---|
1 | SAXN108_0683 | sarA | Transcriptional regulator SarA | Probably activates the development of biofilm by both enhancing the ica operon transcription and suppressing the transcription of either a protein involved in the turnover of PIA/PNAG or a repressor of its synthesis, whose expression would be sigma-B-dependent |
2 | SAXN108_2673 | sbi | Immunoglobulin-binding protein Sbi | Plays a role in the inhibition of both the innate and adaptive immune responses |
3 | SAXN108_1846 | splA | Serine protease SplA | Poorly characterized secreted protein probably involved in virulence |
4 | SAXN108_0774 | saeR | Response regulator transcription factor SaeR | The saeR/S system plays a role in regulating such virulence factors which decrease neutrophil hydrogen peroxide and hypochlorous acid production following S. aureus phagocytosis |
5 | SAXN108_0773 | saeS | Histidine kinase | |
6 | SAXN108_2677 | hlgB | Gamma-hemolysin component B precursor | Toxins that seem to act by forming pores in the membrane of the cell; has a hemolytic and a leukotoxic activity |
7 | SAXN108_2676 | hlgC | Gamma-hemolysin component C precursor |
PIA = polysaccharide intercellular adhesin; PNAG = poly-N-acetyl-β-(1-6)-glucosamine.
Discussion
Panchvalkal-exposed P. aeruginosa appears to suffer from sulfur starvation and nitrosative stress. Compromised nitric oxide (NO) detoxification can render bacteria more susceptible to the NO produced by the host immune system (19). Mutant P. aeruginosa deficient in NO reductase was shown to register a reduced survival rate in NO-producing macrophages (26). NO has a strategic role in the metabolism of microorganisms in natural environments and also during host-pathogen interactions. NO as a signaling molecule is able to influence group behavior in microorganisms. Downregulation of the denitrification pathway can disturb the homeostasis of the bacterial biofilms. NO levels can also affect motility, attachment, and group behavior in bacteria by affecting various signaling pathways involved in the metabolism of 3ʹ,5ʹ-cyclic diguanylic acid (c-di-GMP). Suppressing bacterial detoxification of NO can be an effective anti-pathogenic strategy, as NO is known to modulate several aspects of bacterial physiology, including protection from oxidative stress and antimicrobials, homeostasis of the bacterial biofilm, etc. (27,28,29). From this in silico exercise, nitric oxide reductase (NOR) has emerged as the most important target of Panchvalkal in P. aeruginosa. NOR is one of the important detoxifying enzymes of this pathogen, which is crucial to its ability to withstand nitrosative stress, and has also been reported to be important for virulence expression of this pathogen, and thus can be a plausible potential target for novel anti-virulence agents (19). NOR inhibitors can be expected to compromise the pathogen’s ability to detoxify nitric oxide (NO), not allowing its virulence traits (e.g., biofilm formation, as NO has been indicated to act as a biofilm-dispersal signal) to be expressed fully. NOR inhibitors can be expected to be effective not only against P. aeruginosa but against multiple other pathogens too, as NO is reported to be perceived as a dispersal signal by various gram-negative and gram-positive bacteria (30). This is to say, NOR inhibitors may be expected to have broad-spectrum activity against multiple pathogens. Major function of NOR is to detoxify NO generated by nitrite reductase (NIR). NO is a toxic byproduct of anaerobic respiration in P. aeruginosa. NO-derived nitrosative species can damage DNA and compromise protein function. Intracellular accumulation of NO is likely to be lethal for the pathogen. It can be logically anticipated that P. aeruginosa’s ability to detoxify NO will be compromised under the influence of potent NOR inhibitors like Panchvalkal. Since NO seems to have a broad-spectrum anti-biofilm effect, NOR activity is essential for effective biofilm formation by the pathogens. NOR activity and NO concentration can modulate cellular levels of c-di-GMP, which is a secondary messenger molecule recognized as a key bacterial regulator of multiple processes such as virulence, differentiation, and biofilm formation (31). In the mammalian pathogens, the host’s macrophages are a likely source of NO. NOR expressed by the pathogen provides protection against the host defense mechanism (26). Since NOR activity is known to be important in multiple pathogenic bacteria (e.g., P. aeruginosa, S. aureus, Serratia marcescens) for biofilm formation, virulence expression, combating nitrosative stress, and evading hose defense, NOR seems to be an important target for novel broad-spectrum anti-pathogenic agents. A potential NOR inhibitor besides troubling the pathogen directly may also boost its clearance by the host macrophages (32).
Based on the analysis of differently expressed upregulated genes, sulfur-starved culture of P. aeruginosa can be expected to experience compromised virulence. Upregulation of organic sulfur transport and metabolism genes has been reported in P. aeruginosa facing sodium hypochlorite-induced oxidative stress (33). Two of the upregulated hubs mentioned in Table II are part of tau or ssu gene clusters, which are reported in gram-negative bacteria like Escherichia coli too for being necessary for the utilization of taurine and alkane sulfonates as sulfur sources. Since these genes are exclusively expressed under conditions of sulfate or cysteine starvation (34), one of the multiple effects exerted by Panchvalkal on P. aeruginosa can be said to be sulfur starvation. Upregulation of n-alkane sulfonates or taurine (sources of carbon and organic sulfur) utilization genes in P. aeruginosa suggests that the sulfur in these compounds was used to counter Panchvalkal-induced sulfur starvation, and that the neutrophilic amines and alpha-amino acids formed by catabolization of n-alkane sulfonates may guard the cell against oxidative stress (35). Thus, depriving P. aeruginosa of sulfur can be viewed as a potential anti-virulence strategy.
Among the potential targets identified in S. aureus in this study, first we discuss two such downregulated genes which are common members of two different clusters. Of them, splA is a serine protease, exclusively specific to S. aureus, and thought to have a role in the second invasive stage of the infection (36). Another potential hub sbi is an IgG-binding protein, which has a role in the inhibition of the innate as well as adaptive immune responses. Its secreted form acts as a potent complement inhibitor of the alternative pathway-mediated lysis. sbi helps mediate bacterial evasion of complement via a mechanism called futile fluid-phase consumption (37). Among the remaining potential hubs listed in Table III, SaeR/S two-component system is recognized as a major contributor to S. aureus pathogenesis and neutrophil evasion. SaeR/S also plays a role in regulating such virulence factors which decrease neutrophil hydrogen peroxide and hypochlorous acid production following S. aureus phagocytosis (38). S. aureus escapes from the antimicrobial protein’s neutrophil extracellular traps (NETs), which is dependent on its secreting nuclease (nuc), and the latter in turn is regulated by SaeR/S. The SaeR/S system also modulates neutrophil fate by inhibiting interleukin (IL)-8 production and nuclear factor (NF)-κB activation. SaeR/S deletion mutant of S. aureus was shown to be inferior than its wild-type counterpart in causing programmed neutrophil death (39). The SaeR/S system regulates expression of many important virulence factors in S. aureus, and some of them do appear in our list of important targets such as sbi, hlgB, and hlgC. Thus, inhibiting SaeR/S from sensing its environment can be expected to prevent expression of a multitude of S. aureus virulence factors in response to host signals. hlgB and hlgC are hemolytic proteins, and such proteins are used by many pathogens to fulfill their iron requirement as the concentration of free iron in human serum is much lesser than that required by the bacteria (40). Downregulation of bacterial hemolytic machinery may push them toward iron starvation, thus compromising their fitness for in-host survival. This corroborates well with our earlier report (11) describing reduced hemolytic potential of S. aureus under the influence of Herboheal. Among all the potential hubs identified in Herboheal-exposed S. aureus, only one (sarA) was upregulated, and its upregulation seems to be a response from S. aureus to compensate the Herboheal-induced downregulation of many important virulence traits. For example, sarA regulates expression of ica operon, which is required for biofilm formation in S. aureus. It can be said that S. aureus’s ability to adhere to surfaces and biofilm formation was compromised in the presence of Herboheal as suggested by downregulation of adhesion/biofilm-relevant genes (SaeR/S and sarA), and as an adaptation to such challenge the pathogen is trying to upregulate SarA. This corroborates well with our previous report describing 56% reduced biofilm formation by S. aureus in the presence of Herboheal (11).
This study has identified certain potential hubs in P. aeruginosa (Tabs. I and II) and S. aureus (Tab. III) which should further be investigated for their candidature as potential anti-pathogenic targets. The most suitable targets in bacterial pathogens would be the ones which are absent from their host, as this will allow the criteria of selective toxicity to be satisfied for a newly discovered drug. We did a gene co-occurrence pattern analysis of gene families across genomes (through STRING) with respect to the major hubs identified in each of the pathogens (Tab. IV). Of the 19 hubs identified in either of the pathogen, none was shown to be present in Homo sapiens, and hence drugs causing dysregulation of one or more of these genes in pathogens are less likely to be toxic to humans.
If any target gene is present among multiple pathogens, then it can be considered suitable for a broad-spectrum antibacterial. We analyzed the co-occurrence of identified hubs among some of the important pathogens listed by CDC and WHO. From among those listed in Table IV, atsB, msuE, ssub1, norE, and norB seemed to be present in multiple gram-negative as well as gram-positive pathogens, and thus suitable to be targeted by a broad-spectrum anti-pathogenic discovery program. On the other hand, tauA and nirQ seemed to be present only among gram-negative pathogens. They can prove to be important targets in light of the fact that discovery of novel antimicrobials against gram-negative bacteria is relatively more challenging (41).
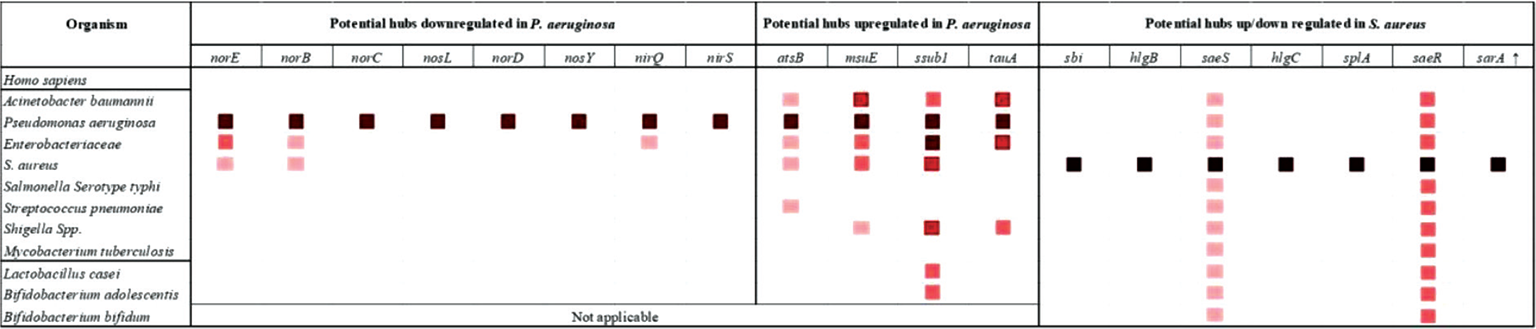
The darker the shade of the squares, higher is the homology between the genes being compared.
One of the issues with conventional antibiotics is that they cannot differentiate between the ‘good’ (symbionts in human microbiome) and ‘bad’ (pathogens) bacteria, and hence their consumption may lead to gut dysbiosis. An ideal antimicrobial agent should target pathogens exclusively without causing gut dysbiosis. In this respect, a target in pathogenic bacteria absent from symbionts of human microbiome will be the most suitable candidate for antibiotic discovery programs. To gain some insight on this front regarding the targets identified by us, we run a gene co-occurrence analysis with some representative ‘good’ bacteria reported to be part of healthy human microbiome. Bifidobacterium species showed presence of no other target except SaeR/S. SaeR/S being widely distributed among bacteria can be considered a valid target; however, an antibacterial agent targeting it may lead to gut dysbiosis too. All downregulated targets in P. aeruginosa were absent from the selected symbionts, which further adds value to their potential candidature as anti-virulence targets. However, atsB and ssub1 appeared to be present in Lactobacillus casei.
Conclusion
This study has identified certain potential targets in two important pathogens. Such in silico studies being predictive in nature, further work is warranted on wet-lab validation of the identified targets. Deletion mutants of the identified hub genes should be assessed for their expected attenuated virulence in appropriate host models. Next-generation pathoblockers targeting any one of these genes may not always be effective as stand-alone therapeutic, and simultaneous targeting of more than one of these genes may be required for an effective therapy. They can also prove to be useful adjuvants to conventional antibiotics allowing use of bactericidal antibiotics at lower concentrations.
Besides indicating generation of nitrosative stress, inducing sulfur starvation, and disturbing regulation of bacterial virulence as potentially effective anti-pathogenic strategies, this study also demonstrates the relevance of the polyherbalism concept of the Traditional Medicine systems, and utility of the network analysis approach in elucidating the multiple modes of anti-pathogenic action exerted by the multicomponent natural extracts.
Acknowledgments
The authors thank Nirma Education and Research Foundation (NERF), Ahmedabad, for infrastructural support; Dr. Palep’s Medical Education and Research Foundation for providing Panchvalkal extract; Pooja Patel and Chinmayi Joshi for help with mining raw data.
Abbreviations
AMR = antimicrobial resistance; DEG = differentially expressed genes; NO = nitric oxide; NOR = nitric oxide reductase; PPI = protein-protein interaction
Disclosures
Conflict of interest: The authors declare no conflict of interest.
Financial support: This research received no specific grant from any funding agency in the public, commercial, or not-for-profit sectors.
Author’s contribution: Conceptualization: VK; Data Curation: FR, SS, JP, NT, GG; Formal Analysis: FR, SS, JP, NT, GG, VK; Funding Acquisition: VK; Investigation: FR, SS, JP, NT, GG; Methodology: VK, GG, NT; Project Administration: VK; Resources: VK; Supervision: VK; Writing – Original Draft: VK, FR; Writing – Review & Editing: VK, GG, NT.
References
- 1. Årdal C, Baraldi E, Theuretzbacher U, et al. Insights into early stage of antibiotic development in small- and medium-sized enterprises: a survey of targets, costs, and durations. J Pharm Policy Pract. 2018;11(1):1-10. CrossRef PubMed
- 2. Årdal C, Balasegaram M, Laxminarayan R, et al. Antibiotic development – economic, regulatory and societal challenges. Nat Rev Microbiol. 2020;18(5):267-274. CrossRef PubMed
- 3. Prasad NK, Seiple IB, Cirz RT, Rosenberg OS. Leaks in the pipeline: a failure analysis of gram-negative antibiotic development from 2010 to 2020. Antimicrob Agents Chemother. 2022;66(5), pp.e00054-22. CrossRef PubMed
- 4. Årdal C, Baraldi E, Busse R, et al. Transferable exclusivity voucher: a flawed incentive to stimulate antibiotic innovation. Lancet. 2023 Feb 9:S0140-6736(23)00282-9. CrossRef PubMed
- 5. Laxminarayan R, Duse A, Wattal C, et al. Antibiotic resistance – the need for global solutions. Lancet Infect Dis. 2013;13(12):1057-1098. CrossRef PubMed
- 6. Cheng G, Dai M, Ahmed S, Hao H, Wang X, Yuan Z. Antimicrobial drugs in fighting against antimicrobial resistance. Front Microbiol. 2016;7:470. CrossRef PubMed
- 7. Silver LL. Challenges of antibacterial discovery. Clin Microbiol Rev. 2011;24(1):71-109. CrossRef PubMed
- 8. Kenny JG, Ward D, Josefsson E, et al. The Staphylococcus aureus response to unsaturated long chain free fatty acids: survival mechanisms and virulence implications. PLoS One. 2009;4(2):e4344. CrossRef PubMed
- 9. Langendonk RF, Neill DR, Fothergill JL. The building blocks of antimicrobial resistance in Pseudomonas aeruginosa: implications for current resistance-breaking therapies. Front Cell Infect Microbiol. 2021;11:665759. CrossRef PubMed
- 10. Joshi C, Patel P, Palep H, Kothari V. Validation of the anti-infective potential of a polyherbal ‘Panchvalkal’ preparation, and elucidation of the molecular basis underlining its efficacy against Pseudomonas aeruginosa. BMC Complement Altern Med. 2019;19(1):19. CrossRef PubMed
- 11. Patel P, Joshi C, Kothari V. Anti-pathogenic efficacy and molecular targets of a polyherbal wound-care formulation (Herboheal) against Staphylococcus aureus. Infect Disord Drug Targets. 2019;19(2):193-206. CrossRef PubMed
- 12. Szklarczyk D, Gable AL, Lyon D, et al. STRING v11: protein-protein association networks with increased coverage, supporting functional discovery in genome-wide experimental datasets. Nucleic Acids Res. 2019;47(D1):D607-D613. CrossRef PubMed
- 13. Chin CH, Chen SH, Wu HH, Ho CW, Ko MT, Lin CY. cytoHubba: identifying hub objects and sub-networks from complex interactome. BMC Syst Biol. 2014;8(Suppl 4):S11. CrossRef PubMed
- 14. Misko TP, Schilling RJ, Salvemini D, Moore WM, Currie MG. A fluorometric assay for the measurement of nitrite in biological samples. Anal Biochem. 1993;214(1):11-16. CrossRef PubMed
- 15. Joshi C, Kothari V, Patel P. Importance of selecting appropriate wavelength, while quantifying growth and production of quorum sensing regulated pigments in bacteria. Recent Pat Biotechnol. 2016;10(2):145-152. CrossRef PubMed
- 16. Barraud N, Hassett DJ, Hwang SH, Rice SA, Kjelleberg S, Webb JS. Involvement of nitric oxide in biofilm dispersal of Pseudomonas aeruginosa. J Bacteriol. 2006;188(21):7344-7353. CrossRef PubMed
- 17. Barnes RJ, Bandi RR, Wong WS, et al. Optimal dosing regimen of nitric oxide donor compounds for the reduction of Pseudomonas aeruginosa biofilm and isolates from wastewater membranes. Biofouling. 2013;29(2):203-212. CrossRef PubMed
- 18. Fida TT, Voordouw J, Ataeian M, et al. Synergy of sodium nitroprusside and nitrate in inhibiting the activity of sulfate reducing bacteria in oil-containing bioreactors. Front Microbiol. 2018;9:981. CrossRef PubMed
- 19. Arai H. Regulation and function of versatile aerobic and anaerobic respiratory metabolism in Pseudomonas aeruginosa. Front Microbiol. 2011;2:103. CrossRef PubMed
- 20. Adamczack J, Hoffmann M, Papke U, et al. NirN protein from Pseudomonas aeruginosa is a novel electron-bifurcating dehydrogenase catalyzing the last step of heme d1 biosynthesis. J Biol Chem. 2014;289(44):30753-30762. CrossRef PubMed
- 21. Dettman JR, Rodrigue N, Aaron SD, Kassen R. Evolutionary genomics of epidemic and nonepidemic strains of Pseudomonas aeruginosa. Proc Natl Acad Sci USA. 2013;110(52):21065-21070. CrossRef PubMed
- 22. Borrero-de Acuña JM, Timmis KN, Jahn M, Jahn D. Protein complex formation during denitrification by Pseudomonas aeruginosa. Microb Biotechnol. 2017;10(6):1523-1534. CrossRef PubMed
- 23. Tralau T, Vuilleumier S, Thibault C, Campbell BJ, Hart CA, Kertesz MA. Transcriptomic analysis of the sulfate starvation response of Pseudomonas aeruginosa. J Bacteriol. 2007;189(19):6743-6750. CrossRef PubMed
- 24. Marquordt C, Fang Q, Will E, Peng J, von Figura K, Dierks T. Posttranslational modification of serine to formylglycine in bacterial sulfatases. Recognition of the modification motif by the iron-sulfur protein AtsB. J Biol Chem. 2003;278(4):2212-2218. CrossRef PubMed
- 25. Hummerjohann J, Laudenbach S, Rétey J, Leisinger T, Kertesz MA. The sulfur-regulated arylsulfatase gene cluster of Pseudomonas aeruginosa, a new member of the cys regulon. J Bacteriol. 2000;182(7):2055-2058. CrossRef PubMed
- 26. Kakishima K, Shiratsuchi A, Taoka A, Nakanishi Y, Fukumori Y. Participation of nitric oxide reductase in survival of Pseudomonas aeruginosa in LPS-activated macrophages. Biochem Biophys Res Commun. 2007;355(2):587-591. CrossRef PubMed
- 27. Gusarov I, Nudler E. NO-mediated cytoprotection: instant adaptation to oxidative stress in bacteria. Proc Natl Acad Sci USA. 2005;102(39):13855-13860. CrossRef PubMed
- 28. Gusarov I, Shatalin K, Starodubtseva M, Nudler E. Endogenous nitric oxide protects bacteria against a wide spectrum of antibiotics. Science. 2009;325(5946):1380-1384. CrossRef PubMed
- 29. Rinaldo S, Giardina G, Mantoni F, Paone A, Cutruzzolà F. Beyond nitrogen metabolism: nitric oxide, cyclic-di-GMP and bacterial biofilms. FEMS Microbiol Lett. 2018;365(6). CrossRef PubMed
- 30. Barraud N, Kelso MJ, Rice SA, Kjelleberg S. Nitric oxide: a key mediator of biofilm dispersal with applications in infectious diseases. Curr Pharm Des. 2015;21(1):31-42. CrossRef PubMed
- 31. Plate L, Marletta MA. Nitric oxide modulates bacterial biofilm formation through a multicomponent cyclic-di-GMP signaling network. Mol. Cell. 2012;449-560. CrossRef
- 32. Schairer DO, Chouake JS, Nosanchuk JD, Friedman AJ. The potential of nitric oxide releasing therapies as antimicrobial agents. Virulence. 2012;3(3):271-279. CrossRef PubMed
- 33. Small DA, Chang W, Toghrol F, Bentley WE. Toxicogenomic analysis of sodium hypochlorite antimicrobial mechanisms in Pseudomonas aeruginosa. Appl Microbiol Biotechnol. 2007;74(1):176-185. CrossRef PubMed
- 34. Eichhorn E, van der Ploeg JR, Leisinger T. Deletion analysis of the Escherichia coli taurine and alkanesulfonate transport systems. J Bacteriol. 2000;182(10):2687-2695. CrossRef PubMed
- 35. Small DA, Chang W, Toghrol F, Bentley WE. Comparative global transcription analysis of sodium hypochlorite, peracetic acid, and hydrogen peroxide on Pseudomonas aeruginosa. Appl Microbiol Biotechnol. 2007;76(5):1093-1105. CrossRef PubMed
- 36. Stec-Niemczyk J, Pustelny K, Kisielewska M, et al. Structural and functional characterization of SplA, an exclusively specific protease of Staphylococcus aureus. Biochem J. 2009;419(3):555-564. CrossRef PubMed
- 37. Burman JD, Leung E, Atkins KL, et al. Interaction of human complement with Sbi, a staphylococcal immunoglobulin-binding protein: indications of a novel mechanism of complement evasion by Staphylococcus aureus. J Biol Chem. 2008;283(25):17579-17593. CrossRef PubMed
- 38. Guerra FE, Addison CB, de Jong NW, et al. Staphylococcus aureus SaeR/S-regulated factors reduce human neutrophil reactive oxygen species production. J Leukoc Biol. 2016;100(5):1005-1010. CrossRef PubMed
- 39. Guerra FE, Borgogna TR, Patel DM, Sward EW, Voyich JM. Epic immune battles of history: neutrophils vs. Staphylococcus aureus. Front Cell Infect Microbiol. 2017;7:286. CrossRef PubMed
- 40. Wilson M. Microbial inhabitants of humans: Their ecology and role in health and disease. Cambridge University Press 2005;15:chap 1.
- 41. Muñoz KA, Hergenrother PJ. Facilitating compound entry as a means to discover antibiotics for gram-negative bacteria. Acc Chem Res. 2021;54(6):1322-1333. CrossRef PubMed