![]() |
Drug Target Insights 2022; 16: 54-68 ISSN 1177-3928 | DOI: 10.33393/dti.2022.2512 ORIGINAL RESEARCH ARTICLE |
![]() |
Exploring the inhibitory mechanisms of indazole compounds against SAH/MTAN-mediated quorum sensing utilizing QSAR and docking
ABSTRACT
The world is under the great threat of antimicrobial resistance (AMR) leading to premature deaths. Microorganisms can produce AMR via quorum sensing mechanisms utilizing S-adenosyl homocysteine/methylthioadenosine nucleosidase (SAH/MTAN) biosynthesis. But there is no specific drug developed to date to stop SAH/MTAN, which is a crucial target for the discovery of anti-quorum sensing compound. It has been shown that indazole compounds cause inhibition of SAH/MTAN-mediated quorum sensing, but the biochemical mechanisms have not yet been explored. Therefore, in this original research, an attempt has been made to explore essential structural features of these compounds by quantitative structure-activity relationship (QSAR) and molecular docking of indazole compounds having inhibition of SAH/MTAN-mediated quorum sensing. The validated QSAR predicted five essential descriptors and molecular docking helps to identify the active binding amino acid residues involved in ligand-receptor interactions that are responsible for producing the quorum sensing inhibitory mechanisms of indazole compounds against SAH/MTAN-mediated AMR.
Keywords: Antimicrobial resistance, Indazole compounds, Molecular docking, QSAR, Quorum sensing, SAH/MTAN
Received: October 26, 2022
Accepted: November 28, 2022
Published online: December 22, 2022
Drug Target Insights - ISSN 1177-3928 - www.aboutscience.eu/dti
© 2022 The Authors. This article is published by AboutScience and licensed under Creative Commons Attribution-NonCommercial 4.0 International (CC BY-NC 4.0).
Commercial use is not permitted and is subject to Publisher’s permissions. Full information is available at www.aboutscience.eu
Introduction
Microbial invasion and its virulence can cause damage to the host cells and antimicrobial resistance (AMR) via quorum sensing (QS) mechanism, which is responsible for the intercellular communication among microbes. As per British government statistical record, it was recently estimated that by 2050 AMR can engulf 10 million lives each year and cause cumulative losses of US$ 100 trillion to world GDP (1). QS represents microbiome population density and utilizes signal molecules responsible for producing drug resistance (2). These signals are chemical autoinducers (AIs) (3). There are two types of AIs such as AI-1 and AI-2. AI-1 is N-acyl homoserine lactone (AHL) whereas AI-2 is furanosyl borate diester (4). The S-adenosyl methionine (SAM) and S-ribosylhomocysteine (SRH) are key components that can be catalyzed by S-adenosyl homocysteine/methylthioadenosine nucleosidase (SAH/MTAN) to produce AI-1 and AI-2 signal molecules. SAH/MTAN is an important enzyme and essential for bacterial metabolism (5). Our lab recently reviewed QS biosynthetic pathway-mediated enzymes responsible for antimicrobial drug resistance. SAM is utilized to synthesize SAH, which is being catalyzed by MTAN to produce SRH. SAH/MTAN is responsible for the recycling of adenine and methionine necessary for bacterial DNA and protein synthesis, respectively (6). SAH/MTAN, a very essential component for creating bacterial virulence, could be an attractive target for the disruption of SAM biosynthesis. Schramm developed some MTAN transition state analogues such as methyl (MT), ethyl (EtT), and butyl (But)-substituted immucillin A and DADMe-immucillin A derivatives such as methyl (MT), ethyl (EtT), butyl (BuT), and PhT (Phenyl) substituted having structural resemblance with MTAN. MT-immucillin A and MT-DADMe-immucillin A were found to be slow-onset tight-binding inhibitors of cellular MTAN activity in Vibrio cholerae and wild-type Escherichia coli (7).
Tedder et al designed and synthesized many SAH/MTAN inhibitors using 6-substituted purine and deaza purines as the core scaffolds. Some of them produced low nM inhibitors with broad-spectrum antimicrobial activity (8). Li et al designed 5-aminoindazole derivatives using structure-guided methods that screened several low-nanomolar inhibitors with broad-spectrum antimicrobial activity to combat SAH/MTAN-mediated QS. X-ray crystal structure of lead compounds cocrystallized with SAH/MTAN obtained from E. coli and other pathogenic bacteria revealed the mode of binding of the inhibitor toward the target site. These cocrystal structures could provide structural information for the design of more active congeneric compounds in the series (9).
But there is hardly any QSAR utilizing theoretical molecular descriptors and docking studies carried out toward these potential congeners. Therefore, an attempt has been made in the present study to explore the biochemical mechanisms of indazole compounds against SAH/MTAN utilizing QSAR and docking tools.
Experimental methods
Activity data
The biological activity data consist of 40 indazole compounds (Tab. I) designed, synthesized, and tested by Li et al (9). These compounds evaluated the biological inhibitory effect of taking SAH/MTAN enzyme expressed on the full-length E. coli pfs gene having high conservation on the bacterial species. The SAH/MTAN is the key target for the production of AHL-mediated AI-1 and SAH-mediated AI-2, which are the building blocks for the synthesis of the QS AIs (9). The enzyme inhibitory activities have been measured in terms of Ki measuring the affinity of the compound to bind the active cavity of SAH/MTAN. A negative logarithm of these Ki values (pKi) has been done for data reduction and taken as a dependent variable whereas molecular structural descriptor has been computed as independent variables for these compounds.
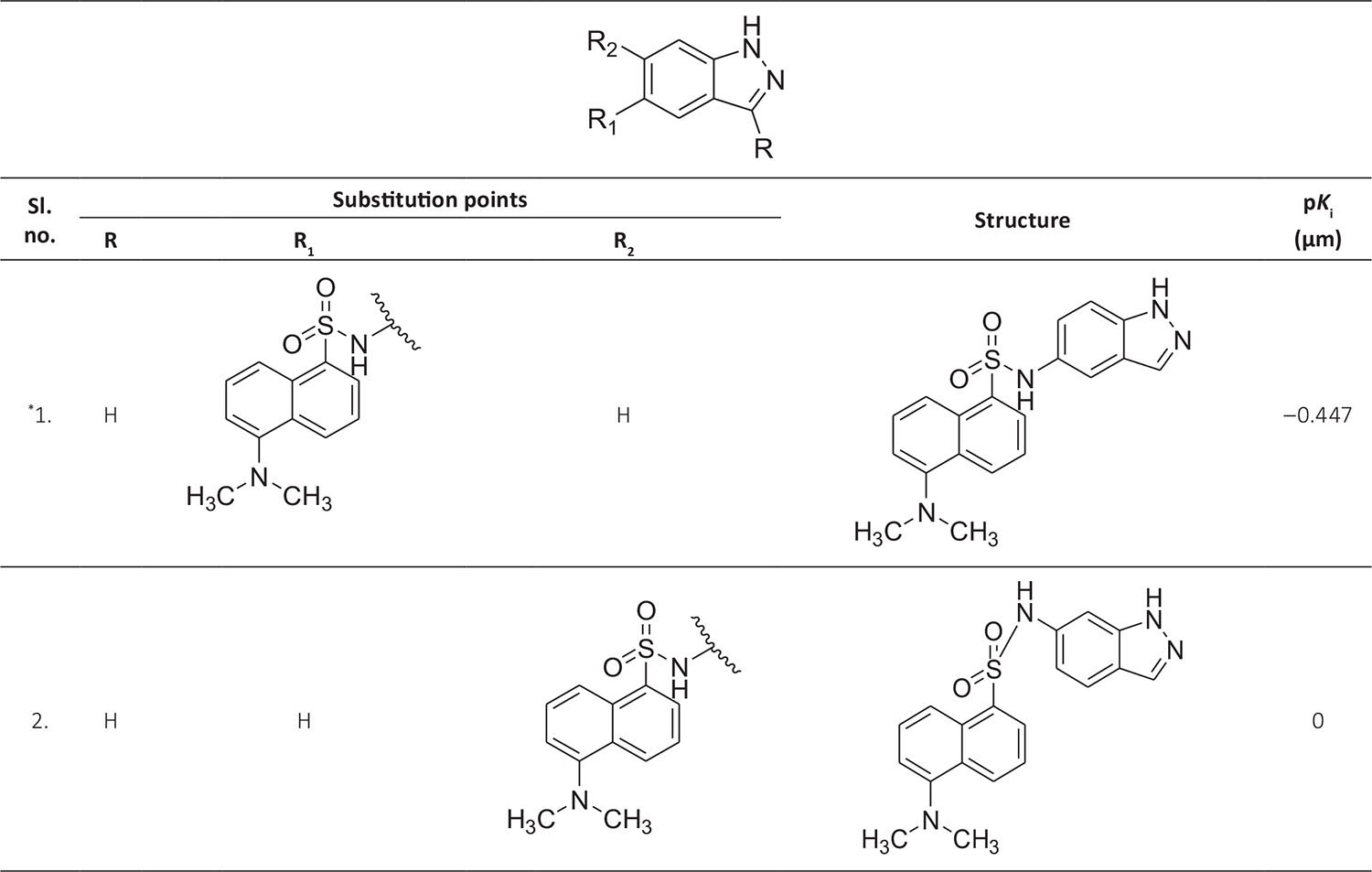
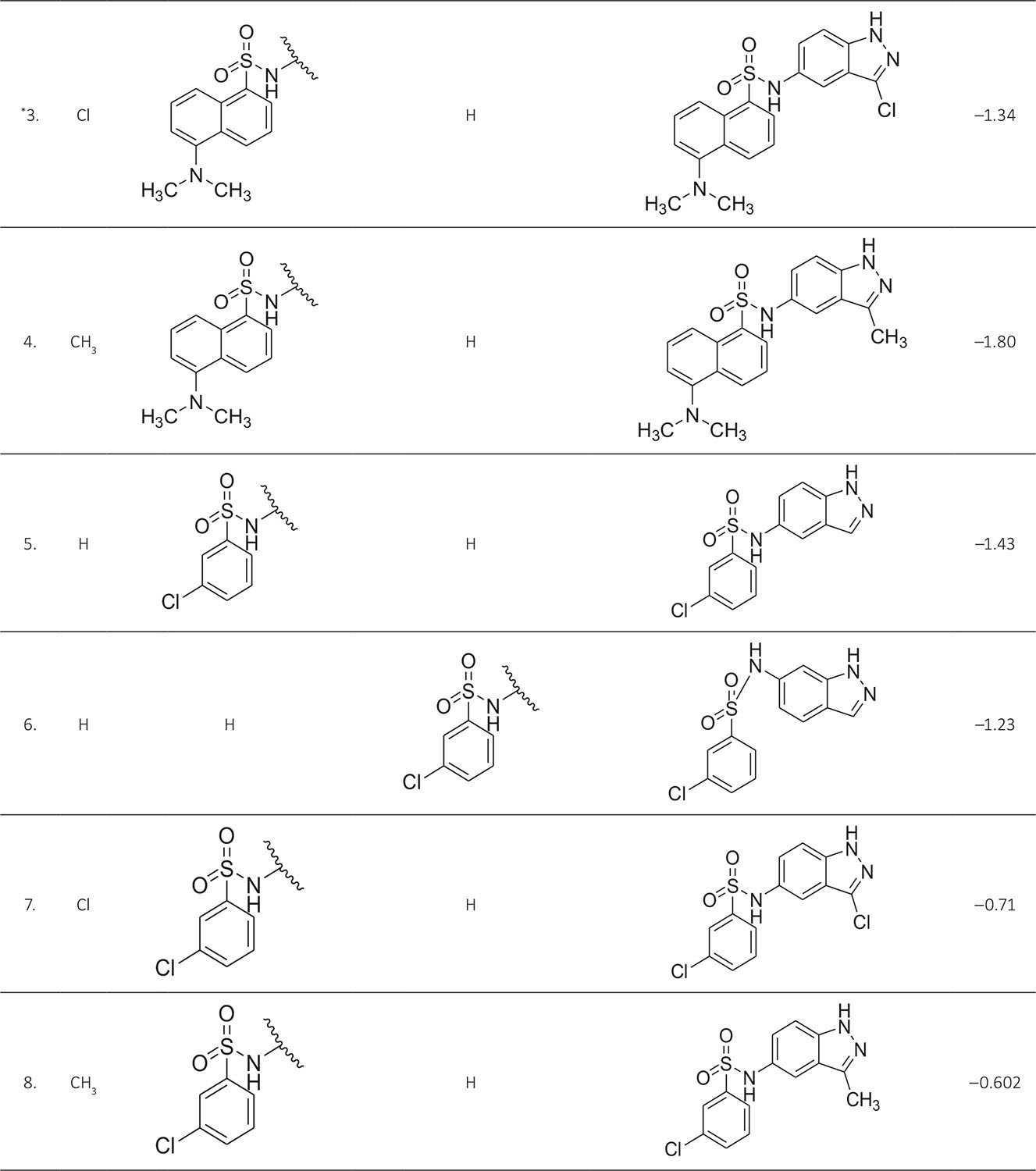
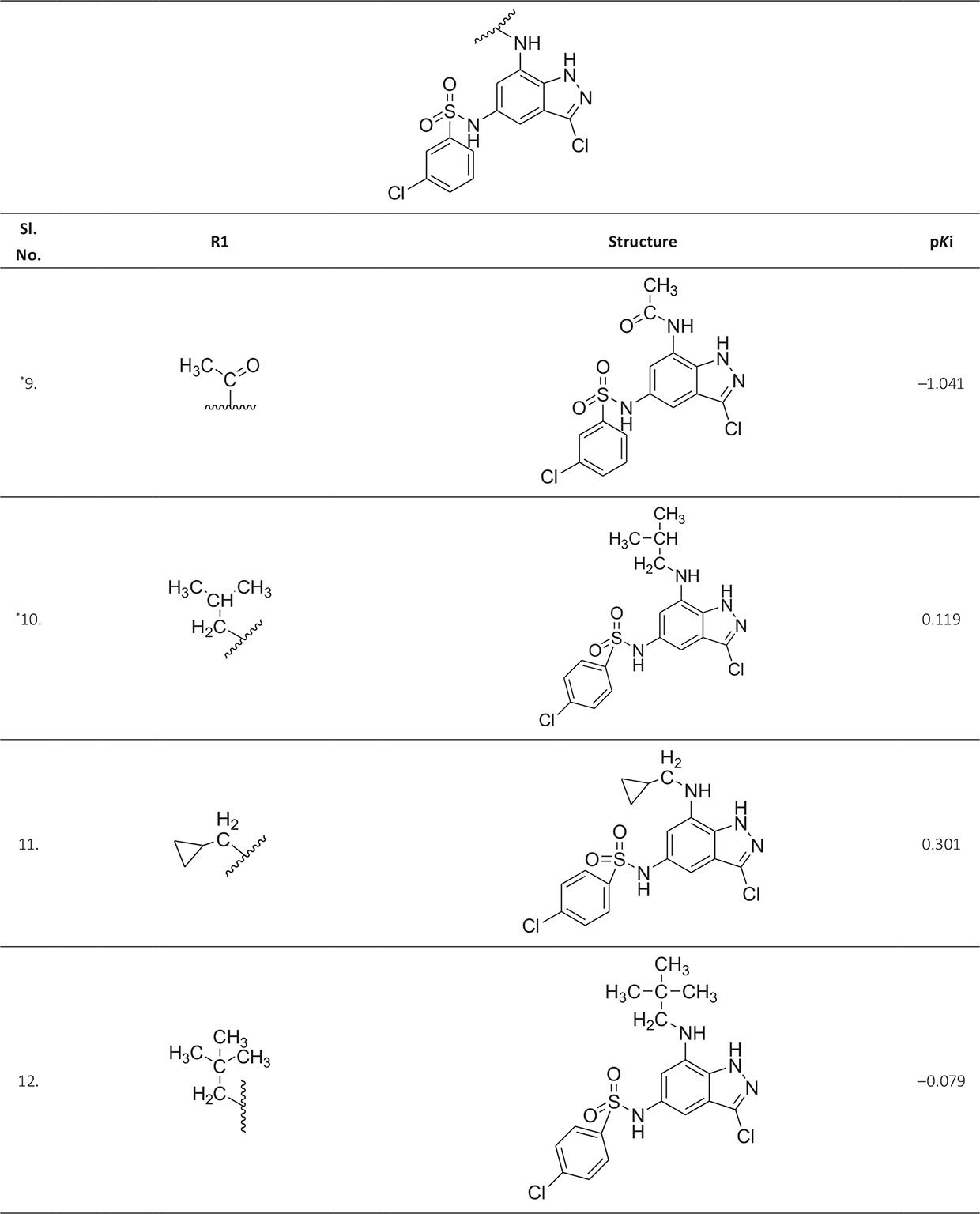
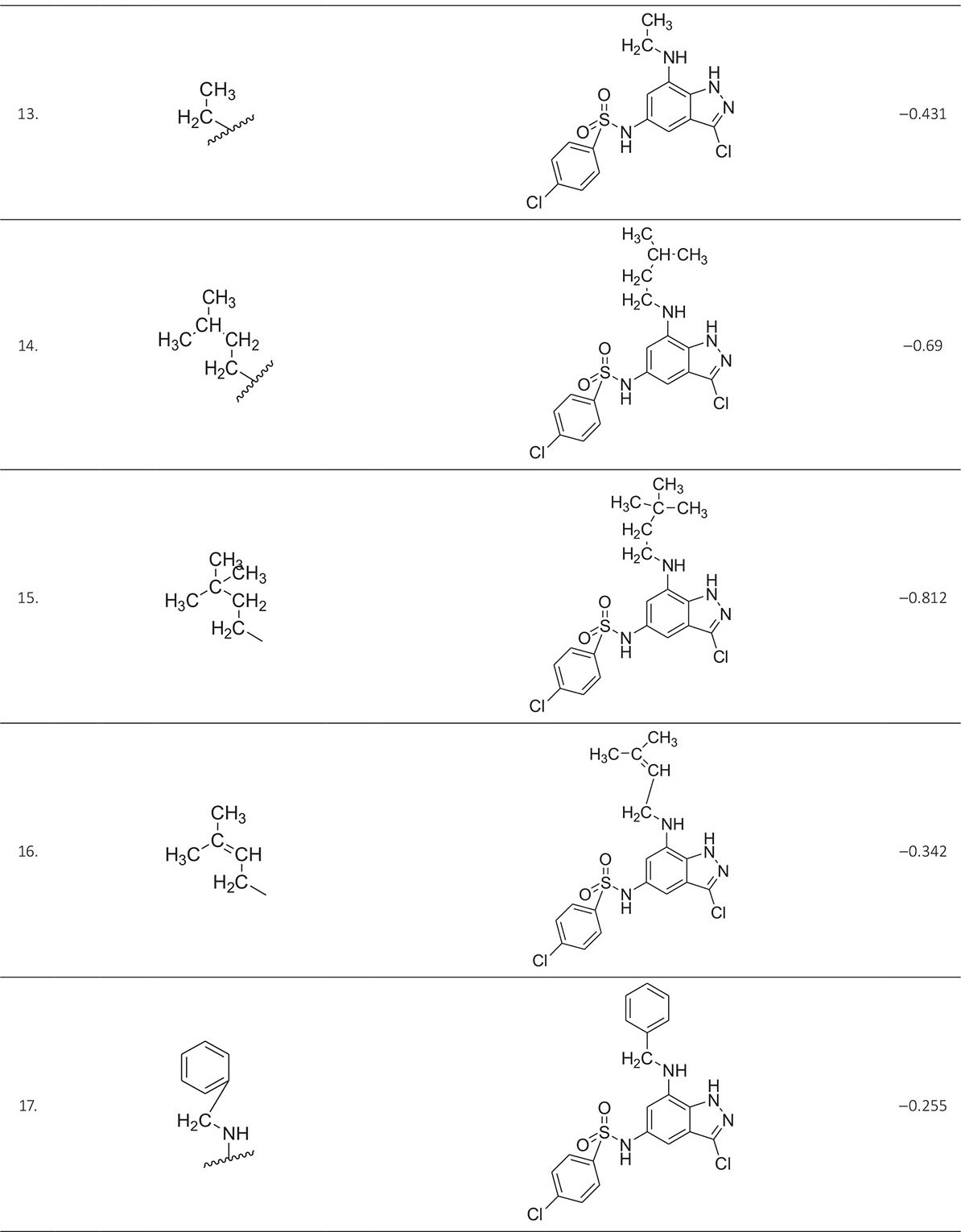
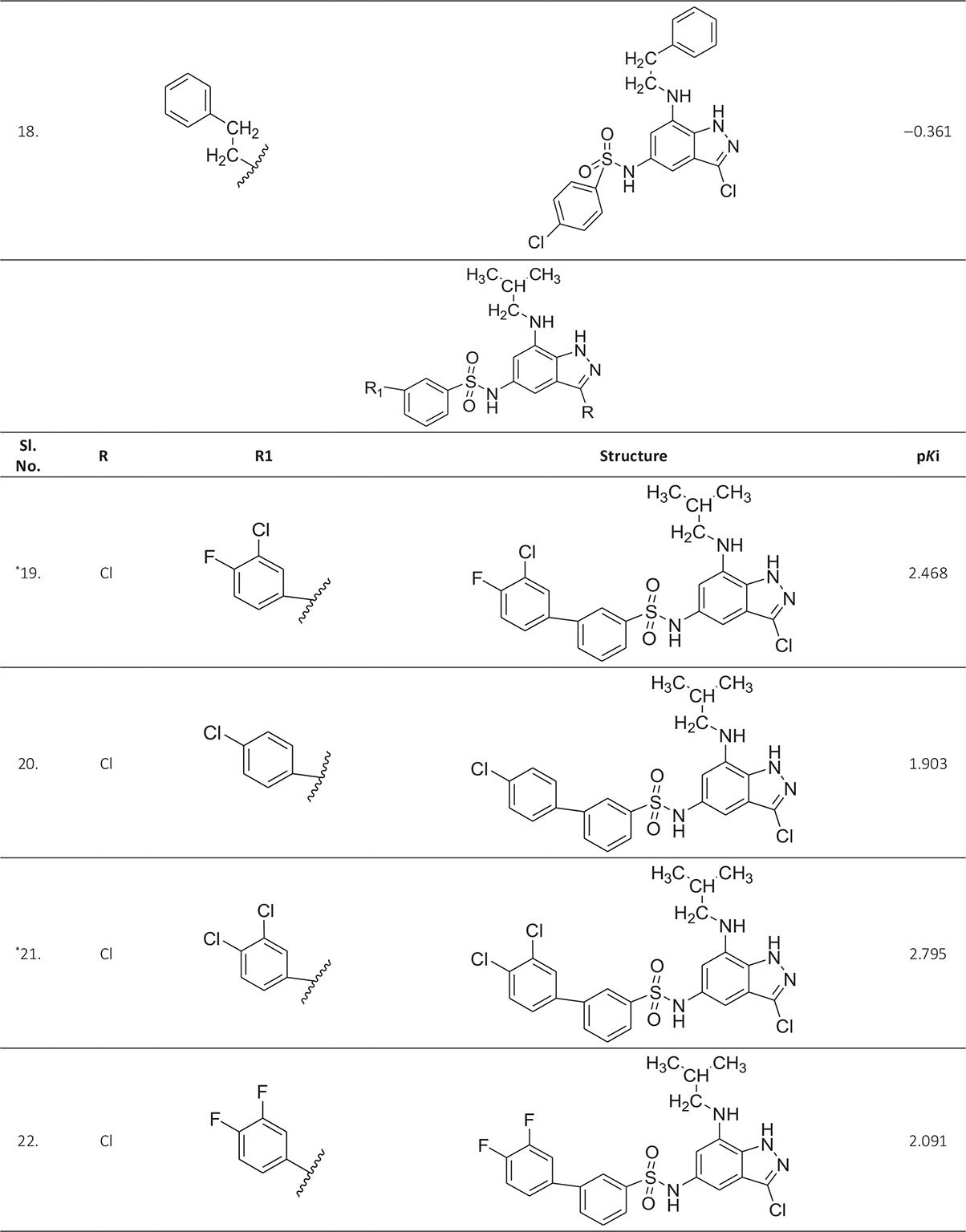
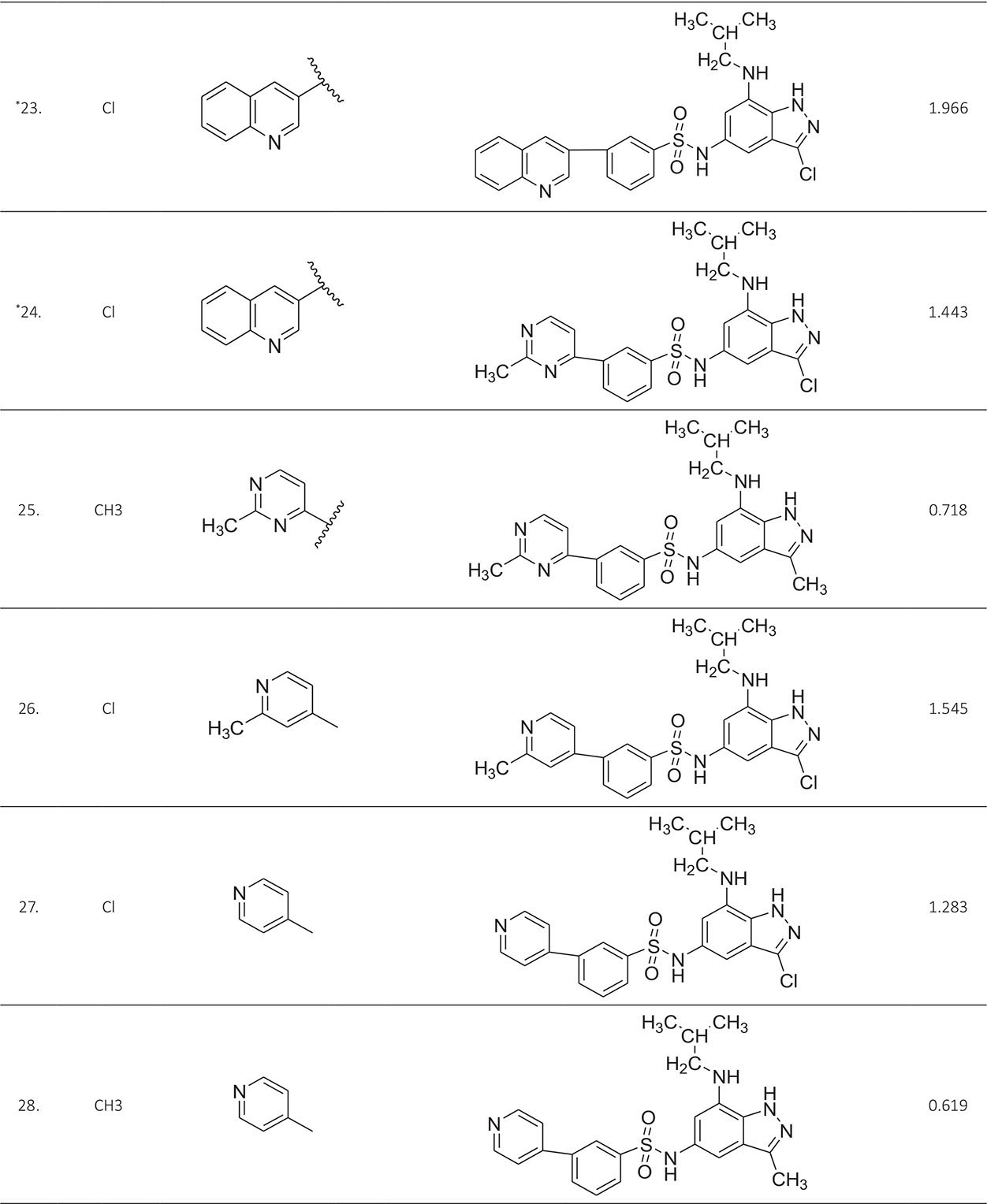
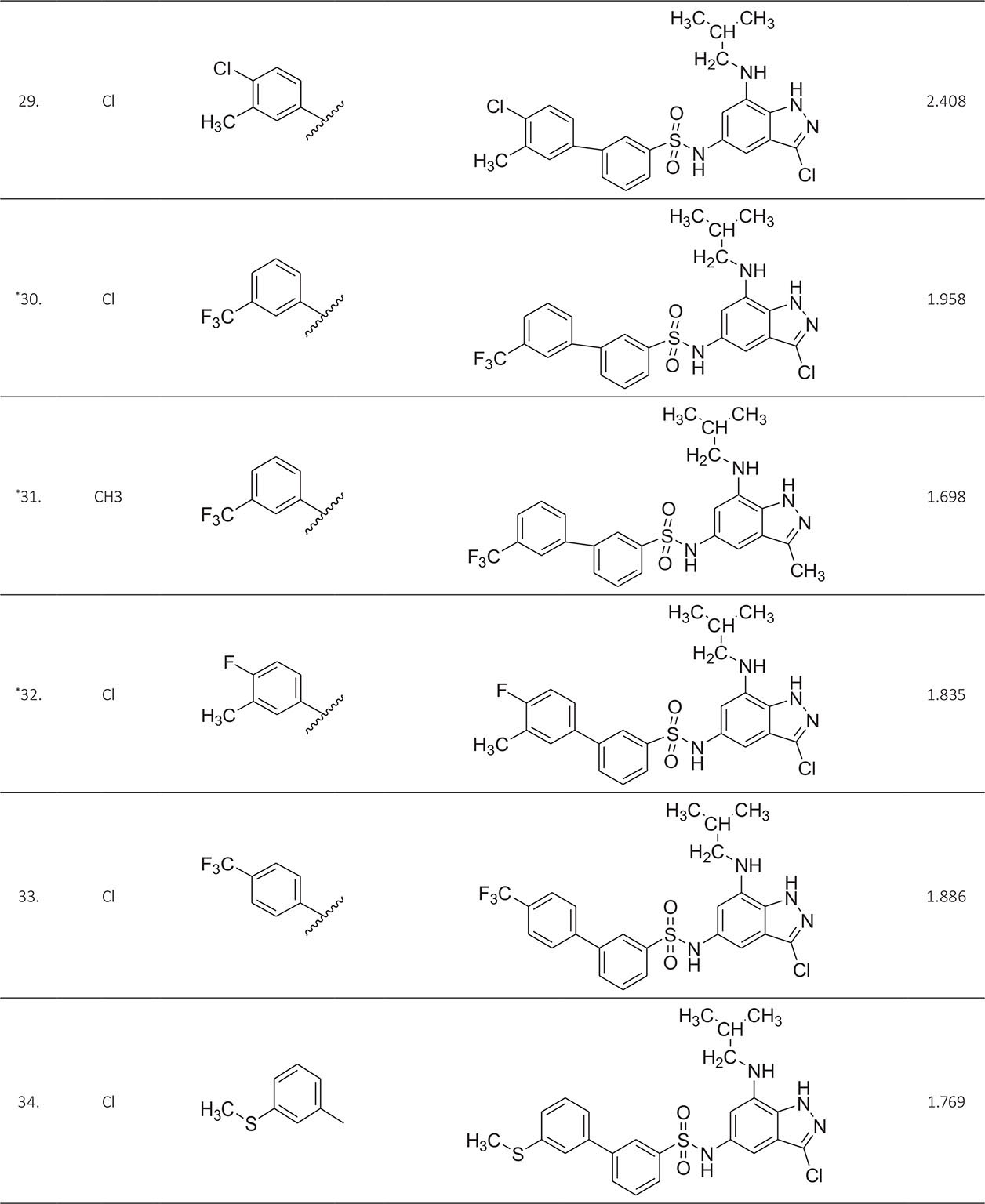
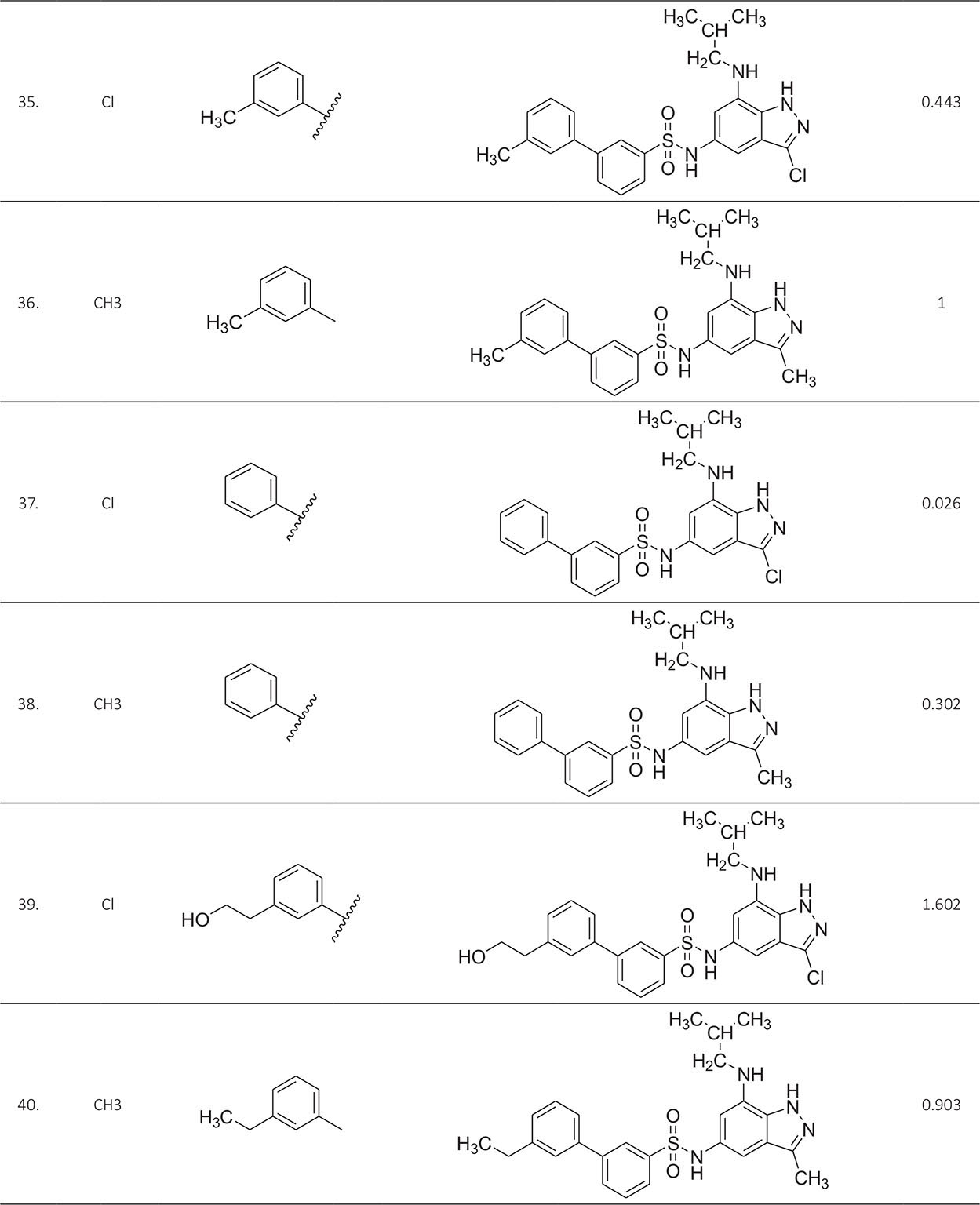
* Test compounds.
Methods of computation
Structure computation and molecular optimization
The two-dimensional structures of 40 indazole compounds were drawn using Chemdraw 8.0 software. These structures were transformed into 3D files, which are minimized using the MM2 force field. The molecular energy minimization is carried out taking the convergence criterion and dielectric constant of 0.01 Kcal/mol and 1.0 respectively (10) utilizing the Chem3D Ultra window (11).
Molecular descriptors computation
All the three-dimensional mol files were incorporated into PaDEL Descriptor Computation software for the computation of structural descriptors. PaDEL is a freeware for the calculation of molecular properties (12). A total number of 1,875 2D and 3D descriptors were calculated and before the model generation, this descriptor data had been reduced to 1,055 (Tab. II). Perfectly constant and highly intercorrelated descriptors were removed taking variance and correlation coefficient cut-off values of 0.0001 and 0.9 using the V-WSP algorithm of NanoBridges software (13). The reduced descriptor data set has been used for the development of QSAR models.
ALogP, ALogp2, AMR, apol, naAromAtom, nAtom, nHeavyAtom, nH, nC, nN, nO, nS, nF, nCl, nX, ATS0m, ATS1m, ATS2m, ATS3m, ATS4m, ATS5m, ATS6m, ATS7m, ATS8m, ATS2v, ATS4v, ATS6v, ATS7v, ATS8v, ATS0e, ATS3e, ATS4e, ATS5e, ATS6e, ATS7e, ATS8e, ATS0p, ATS3p, ATS5p, ATS0s, ATS1s, ATS2s, ATS3s, ATS4s, ATS5s, ATS6s, ATS7s, ATS8s, AATS0m, AATS1m, AATS2m, AATS3m, AATS4m, AATS5m, AATS6m, AATS7m, AATS8m, AATS0v, AATS1v, AATS2v, AATS3v, AATS4v, AATS5v, AATS6v, AATS7v, AATS8v, AATS0e, AATS1e, AATS2e, AATS3e, AATS4e, AATS5e, AATS6e, AATS7e, AATS8e, AATS0p, AATS1p, AATS2p, AATS3p, AATS4p, AATS5p, AATS6p, AATS7p, AATS8p, AATS0i, AATS1i, AATS2i, AATS3i, AATS4i, AATS5i, AATS6i, AATS7i, AATS8i, AATS0s, AATS1s, AATS2s, AATS3s, AATS4s, AATS5s, AATS6s, AATS7s, AATS8s, ATSC0c, ATSC1c, ATSC2c, ATSC3c, ATSC4c, ATSC5c, ATSC6c, ATSC7c, ATSC8c, ATSC0m, ATSC1m, ATSC2m, ATSC3m, ATSC4m, ATSC5m, ATSC6m, ATSC7m, ATSC8m, ATSC0v, ATSC1v, ATSC2v, ATSC3v, ATSC4v, ATSC5v, ATSC6v, ATSC7v, ATSC8v, ATSC0e, ATSC1e, ATSC2e, ATSC3e, ATSC4e, ATSC5e, ATSC6e, ATSC7e, ATSC8e, ATSC0p, ATSC1p, ATSC2p, ATSC3p, ATSC4p, ATSC5p, ATSC6p, ATSC7p, ATSC8p, ATSC0i, ATSC1i, ATSC2i, ATSC3i, ATSC4i, ATSC5i, ATSC6i, ATSC7i, ATSC8i, ATSC0s, ATSC1s, ATSC2s, ATSC3s, ATSC4s, ATSC5s, ATSC6s, ATSC7s, ATSC8s, AATSC0m, AATSC1m, AATSC2m, AATSC3m, AATSC4m, AATSC5m, AATSC6m, AATSC7m, AATSC8m, AATSC0v, AATSC1v, AATSC2v, AATSC3v, AATSC4v, AATSC5v, AATSC6v, AATSC7v, AATSC8v, AATSC0e, AATSC2e, AATSC6e, AATSC7e, AATSC0p, AATSC2p, AATSC3p, AATSC4p, AATSC5p, AATSC6p, AATSC7p, AATSC8p, AATSC0i, AATSC1i, AATSC2i, AATSC3i, AATSC4i, AATSC5i, AATSC6i, AATSC7i, AATSC8i, AATSC0s, AATSC1s, AATSC2s, AATSC3s, AATSC4s, AATSC5s, AATSC6s, AATSC7s, AATSC8s, MATS1c, MATS2c, MATS3c, MATS4c, MATS5c, MATS6c, MATS7c, MATS8c, MATS1m, MATS2m, MATS3m, MATS4m, MATS5m, MATS6m, MATS7m, MATS8m, MATS1e, MATS2e, MATS3e, MATS4e, MATS5e, MATS6e, MATS7e, MATS8e, MATS1p, MATS2i, MATS3i, MATS5i, MATS6i, MATS7i, MATS8i, MATS1s, MATS2s, MATS3s, MATS4s, MATS5s, MATS6s, MATS7s, MATS8s, GATS1c, GATS2c, GATS3c, GATS4c, GATS5c, GATS6c, GATS7c, GATS8c, GATS1m, GATS2m, GATS3m, GATS4m, GATS5m, GATS6m, GATS7m, GATS8m, GATS1v, GATS2v, GATS3v, GATS4v, GATS5v, GATS6v, GATS7v, GATS8v, GATS1e, GATS2e, GATS3e, GATS4e , GATS5e, GATS6e, GATS7e, GATS8e, GATS1p, GATS2p, GATS3p, GATS4p, GATS5p, GATS6p, GATS7p, GATS8p, GATS1i, GATS2i, GATS3i, GATS4i, GATS5i, GATS6i, GATS7i, GATS8i, GATS1s, GATS2s, GATS3s, GATS4s, GATS5s, GATS6s, GATS7s, GATS8s, SpAbs_DzZ, SpMAD_DzZ, SM1_DzZ, VE1_DzZ, VE3_DzZ, VR1_DzZ, VR3_DzZ, VR1_Dzm, VR2_Dzm, VR3_Dzm, SM1_Dzv, VE1_Dzv, VE3_Dzv, VR1_Dzv, VR2_Dzv, VR3_Dzv, SM1_Dze, VE1_Dze, VE3_Dze, VR1_Dze, VR3_Dze, SpAbs_Dzp, SpMAD_Dzp, SM1_Dzp, VE1_Dzp, VE3_Dzp, VR1_Dzp, VR3_Dzp, SM1_Dzi, VE1_Dzi, VE3_Dzi, VR1_Dzi, VR3_Dzi, SpAbs_Dzs, SpMAD_Dzs, SM1_Dzs, VE1_Dzs, VE3_Dzs, VR1_Dzs, VR2_Dzs, VR3_Dzs, BCUTw-1l, BCUTw-1h, BCUTc-1l, BCUTc-1h, BCUTp-1l, BCUTp-1h, nBondsS2, nBondsS3, nBondsD, nBondsD2, nBondsM bpol, SpMax2_Bhm, SpMax3_Bhm, SpMax4_Bhm, SpMax5_Bhm, SpMax6_Bhm, SpMax7_Bhm, SpMax8_Bhm, SpMin1_Bhm, SpMin2_Bhm, SpMin3_Bhm, SpMin4_Bhm, SpMin5_Bhm, SpMin6_Bhm, SpMin7_Bhm, SpMin8_Bhm, SpMax1_Bhv, SpMax2_Bhv, SpMax3_Bhv, SpMax4_Bhv, SpMax5_Bhv, SpMax6_Bhv, SpMax7_Bhv, SpMax8_Bhv, SpMin1_Bhv, SpMin2_Bhv, SpMin3_Bhv, SpMin4_Bhv, SpMin5_Bhv, SpMin6_Bhv, SpMin7_Bhv, SpMin8_Bhv, SpMax1_Bhe, SpMax2_Bhe, SpMax3_Bhe, SpMax4_Bhe, SpMax6_Bhe, SpMax7_Bhe, SpMax8_Bhe, SpMin1_Bhe, SpMin2_Bhe, SpMin3_Bhe, SpMin4_Bhe, SpMin5_Bhe, SpMin6_Bhe, SpMin7_Bhe, SpMin8_Bhe, SpMax1_Bhp, SpMax2_Bhp, SpMax3_Bhp, SpMax4_Bhp, SpMax7_Bhp, SpMin1_Bhp, SpMin2_Bhp, SpMin3_Bhp, SpMin4_Bhp, SpMin7_Bhp, SpMin8_Bhp, SpMax2_Bhi, SpMax3_Bhi, SpMax4_Bhi, SpMax5_Bhi, SpMax8_Bhi, SpMin2_Bhi, SpMin3_Bhi, SpMin4_Bhi, SpMin5_Bhi, SpMin7_Bhi, SpMax1_Bhs, SpMax2_Bhs, SpMax3_Bhs, SpMax4_Bhs, SpMax5_Bhs, SpMax6_Bhs, SpMax7_Bhs, SpMax8_Bhs, SpMin1_Bhs, SpMin2_Bhs, SpMin3_Bhs, SpMin4_Bhs, SpMin5_Bhs, SpMin6_Bhs, SpMin7_Bhs, SpMin8_Bhs, C1SP2, C2SP2, C3SP2, C1SP3, C2SP3, C3SP3, C4SP3, SCH-3, SCH-6, SCH-7, VCH-6, VCH-7, SC-3, SC-4, SC-5, SC-6, VC-3, VC-5, SPC-4,SPC-5, SPC-6, VPC-4, VPC-5, VPC-6, SP-2, SP-3, SP-4, SP-6, SP-7, VP-0, VP-2, VP-3, VP-4, VP-5, VP-6, VP-7, AVP-0, AVP-1, AVP-2, Mare, Mi, CrippenLogP, SpMax_Dt, SpMAD_Dt, VE1_Dt, VE3_Dt, VR1_Dt, VR2_Dt, VR3_Dt, ECCEN, nHBd, nHBa, nwHBa, nHBint2, nHBint3, nHBint4, nHBint5, nHBint6, nHBint7, nHBint8, nHBint9, nHBint10, nHsOH, nHssNH, nHdsCH, nHaaCH, nHCsats, nHCsatu, nsCH3, nssCH2, naasC, naaaC, nssssC, naaN, nsssN, SHBd, SHBa, SwHBa, SHBint2, SHBint3, SHBint4, SHBint5, SHBint6, SHBint7, SHBint8, SHBint9, SHBint10, SHssNH, SHaaNH, SHaaCH, SHCsats, SssCH2, SaaCH, SsssCH, SdssC, SaasC, SaaaC, SssNH, SaaNH, SdO, SddssS, SsCl, minHBd, minHBa, minwHBa, minHBint2, minHBint3, minHBint5, minHBint6, minHBint9, minHBint10, minHaaCH, minHCsats, minHCsatu, minHother, minsCH3, minssCH2, minaaCH, minaasC, minaaaC, minssNH, minaaN, mindO, minsF, minsCl, maxHBd, maxHBa, maxwHBa, maxHBint2, maxHBint3, maxHBint5, maxHBint6, maxHBint10, maxHssNH, maxHaaCH, maxHCsats, maxsCH3, maxssCH2, maxaaCH, maxaasC, maxaaaC, maxssssC, maxssNH, maxaaN, maxdO, maxsCl, sumI, meanI, hmax, LipoaffinityIndex, DELS, MAXDN2, DELS2, ETA_Alpha, ETA_Epsilon_1, ETA_Epsilon_2, ETA_Epsilon_4, ETA_Epsilon_5, ETA_dEpsilon_B, ETA_Psi_1, ETA_Shape_P, ETA_Shape_Y, ETA_Shape_X, ETA_Beta, ETA_BetaP, ETA_Beta_s, ETA_BetaP_s, ETA_Beta_ns, ETA_BetaP_ns, ETA_dBeta, ETA_dBetaP, ETA_Beta_ns_d, ETA_BetaP_ns_d, ETA_Eta, ETA_EtaP, ETA_Eta_F, ETA_EtaP_F, ETA_Eta_L, ETA_EtaP_L, ETA_Eta_F_L, ETA_EtaP_F_L, ETA_Eta_B, ETA_Eta_B_RC, FMF, fragC, nHBAcc, nHBAcc2, nHBAcc3, HybRatio, IC0, IC1, IC2, IC3, IC4, IC5, TIC0, TIC1, TIC2, TIC3, SIC0, SIC1, SIC2, SIC3, SIC4, SIC5, CIC0, CIC1, CIC2, CIC3, CIC4, BIC1, BIC2, BIC3, BIC4, BIC5, MIC0, MIC1, MIC2, MIC3, MIC4, ZMIC0, ZMIC1, ZMIC2, ZMIC3, ZMIC4, ZMIC5, Kier1, Kier2, Kier3, nAtomLC, nAtomP, nAtomLAC, MLogP, MDEC-11, MDEC-12, MDEC-13, MDEC-22, MDEC-23, MDEC-24, MDEC-33, MDEC-34, MDEO-11, MDEN-22, MLFER_A, MLFER_BH, MLFER_S, MLFER_E, MLFER_L, MPC2, MPC3, MPC8, MPC10, piPC1, piPC3, piPC5, piPC6, piPC10, R_TpiPCTPC, PetitjeanNumber, nRing, n6Ring, nTRing, nHeteroRing, nF10HeteroRing, nRotB, RotBFrac, nRotBt, RotBtFrac, LipinskiFailures, topoRadius, topoDiameter, GGI1, GGI2, GGI3, GGI4, GGI5, GGI6, GGI7, GGI8 , GGI9, GGI10, JGI1, JGT, VE1_D, VE3_D, VR1_D VR3_D, TopoPSA, MWC3, MWC6, MWC10, SRW7, SRW9, AMW, WTPT-2, WTPT-3, WPATH, XLogP, TDB1u, TDB2u, TDB3u, TDB4u, TDB5u, TDB6u, TDB7u, TDB8u, TDB9u, TDB10u, TDB6m, TDB7m, TDB8m, TDB9m, TDB10m, TDB1v, TDB3v, TDB4v, TDB5v, TDB6v, TDB7v, TDB8v, TDB9v, TDB10v, TDB1e, TDB2e, TDB3e, TDB4e, TDB5e, TDB6e, TDB7e, TDB8e, TDB9e, TDB10e, TDB1p, TDB3p, TDB4p, TDB5p, TDB6p, TDB7p, TDB8p, TDB9p, TDB10p, TDB1i, TDB2i, TDB3i, TDB4i, TDB5i, TDB6i, TDB7i, TDB8i, TDB9i, TDB10i, TDB1s, TDB3s, TDB5s, TDB6s, TDB7s, TDB8s, TDB9s, TDB10s, TDB1r, TDB2r, TDB3r, TDB4r, TDB5r, TDB6r, TDB7r, TDB8r ,TDB9r, TDB10r, PPSA-1, PPSA-2, PPSA-3, PNSA-1, PNSA-2, PNSA-3, DPSA-1, DPSA-2, DPSA-3, FPSA-1, FPSA-2, FNSA-2, FNSA-3, WPSA-1, WPSA-2, WPSA-3, WNSA-1, WNSA-2, WNSA-3, RPCG, RNCG, RPCS, RNCS, THSA, TPSA, RHSA, GRAV-1, GRAVH-3, GRAV-4, LOBMAX, LOBMIN, MOMI-X, MOMI-Y, MOMI-Z, MOMI-XY, MOMI-XZ, MOMI-R, geomRadius, geomDiameter, geomShape, RDF10u, RDF15u, RDF20u, RDF25u, RDF30u, RDF35u, RDF40u, RDF45u, RDF50u, RDF55u, RDF60u, RDF65u, RDF70u, RDF75u, RDF80u, RDF85u, RDF90u, RDF95u, RDF100u, RDF105u, RDF110u, RDF115u, RDF120u, RDF125u, RDF130u, RDF135u, RDF140u, RDF145u, RDF150u, RDF155u, RDF15m, RDF20m, RDF25m, RDF30m, RDF35m, RDF40m, RDF45m, RDF50m, RDF55m, RDF60m, RDF65m, RDF70m, RDF75m, RDF80m, RDF85m, RDF90m, RDF95m, RDF100m, RDF105m, RDF110m, RDF115m, RDF120m, RDF125m, RDF130m, RDF135m, RDF140m, RDF145m, RDF150m, RDF155m, RDF20v, RDF25v, RDF30v, RDF35v, RDF40v, RDF45v, RDF50v, RDF55v, RDF60v, RDF65v, RDF70v, RDF75v, RDF80v, RDF85v, RDF90v, RDF95v, RDF100v, RDF105v, RDF110v, RDF115v, RDF120v, RDF125v, RDF130v, RDF135v, RDF140v, RDF145v, RDF150v, RDF155v, RDF30e, RDF35e, RDF70e, RDF80e, RDF95e, RDF100e, RDF155e, RDF15p, RDF20p, RDF30p, RDF35p, RDF40p, RDF45p, RDF50p,RDF60p, RDF65p, RDF70p, RDF75p, RDF80p, RDF85p, RDF90p, RDF95p, RDF100p, RDF115p, RDF130p, RDF135p, RDF140p, RDF145p, RDF150p, RDF155p, RDF30i, RDF65i, RDF10s, RDF15s, RDF20s, RDF25s, RDF30s, RDF35s, RDF40s, RDF45s, RDF50s, RDF55s, RDF60s, RDF65s, RDF70s, RDF75s, RDF80s, RDF85s, RDF90s, RDF95s, RDF100s, RDF105s, RDF110s, RDF115s, RDF120s, RDF125s, RDF130s, RDF135s, RDF140s, RDF145s, RDF150s, RDF155s, L1u, L2u, L3u, P1u, P2u, E1u, E2u, E3u, Tu, Au, Vu, Du, L1m, L2m, L3m, P1m, P2m, E1m, E2m, E3m, Tm, Am, Vm, Dm, L1v, L2v, L3v, P1v, P2v, E1v, E2v, E3v, Tv, Av, Vv, Dv, P2e, E1e, E2e, E3e, De, L1p, P1p, P2p, E1p, E2p, E3p, Dp, E1i, E2i, Di, E1s, E2s. |
QSAR model generation followed by validation
A number of QSAR models have been generated for the deliberated indazole compounds utilizing various sets of a combination of 2D and 3D descriptors using genetic algorithm coupled multiple linear regression (GA-MLR) methods (14) based on the theory of mutation and crossover of the parents’ genes to generate the new solutions, taking the most appropriate transformations of the independent variables incorporated in the NanoBridges software (15,16). A population of 100 different random combinations of the structural descriptors is generated taking default parameters as set in the NanoBridges software (17). The impact of these indazole compounds’ computed descriptors on SAH/MTAN inhibitory activities has been shown through QSAR model development by considering each parent combination of descriptors for the entire data set using MLR. The entire data set was divided randomly into test and training sets before QSAR modeling. The developed models were validated statistically. The validation parameters are denoted by R2 (R is the square root of multiple R-square for regression), Q2 (cross-validated r2) values for the training set, whereas external validation was carried out by calculating predictive R2 (R2pred) and the standard error of estimation (SEE) represents standard deviation measured by the error mean square, which expresses the variation of the residuals or the variation about the regression line (18). Further, the external predictability of the generated QSAR models was scrutinized by calculating modified r2 (r2m), average modified r2 (rm2), and delta modified r2 (∆r2m) respectively (19). The best training model is composed of 72.5% and the test set consists of 27.5% of the total data. The test compounds have been marked in Table I with an asterisk.
Ligand docking
All optimized ligands were docked into the receptor active cavity using molecular docking, which is a powerful structure-based drug discovery simulation for the identification of ligand-receptor complexes having minimal interaction energy. The energy of interactions between ligand and protein was calculated in terms of the score, which can predict the affinity of the compound toward active binding (20,21). The crystal structure of E. coli SAH/MTAN (PDB ID: 1JYS) in complex with adenine cocrystal was selected as a receptor for in silico molecular docking studies (22).
The protein was downloaded and prepared by removing water molecules, and hydrogen atoms in the H-depleted target molecule were added. Grid points were generated surrounding the cocrystallized ligand bound with the active cavity of the target. This cocrystallized molecule is considered a reference to make the binding site for the ligand X-ray group. A flexible docking module was incorporated in ArgusLab 4.0.1, which is a very powerful docking simulation freeware (23,24,25). In the present docking simulation, the ligand is freely rotated inside the target cavity to generate multiple 150 conformers that can produce many docked complex poses considering grid resolution (angle) of 0.4 degrees as the default value. The term pose usually designates the specific set of coordinates of a docked ligand. The coordinates of conformation will change concerning this docked pose. The ligand is docked inside the target of the active site, which is well-kept within the grid box. The best complex pose with minimal interaction energy has been taken into consideration for a better explanation of the mode of interaction between the ligand and active amino acid residues of the receptor protein (26).
Results and discussion
QSAR modeling
In the present study, QSAR modeling of indazole compounds having inhibitory activities against SAH/MTAN-mediated QS has been carried out utilizing a different combination of 2D and 3D structural descriptors. The impact of the different classes of computed descriptors on SAH/MTAN inhibitory activities of these compounds has been discussed by the development of optimal training QSAR model formulated by 72.5% of total data and the remaining 27.5% of total data is used as a test set marked by an asterisk. These model parameters have been expressed as R2 and Q2 (cross-validated r2) values for the training set while the external model validation significance is carried out by calculating predictive R2 (R2pred), the SEE and modified r2 (r2m) given in Table III.
It was shown that Equation [1] can produce an explained variance of 71.4% and an internal predicted variance of 55.6% of the observed data. For a predictive QSAR model, the value of R2 should be more than 0.6 (27). The external model validation parameters such as R2pred, r2m, and SEE are given as 0.151, 0.112, and 0.660, which do not produce significant predictability because R2pred and r2m must be greater than 0.5, while the SEE values should be less than 0.5 to have a significant model (28).
Therefore, outlier analyses have been carried out by testing of applicability domain of the training QSAR model, which determines its acceptance as per the Organization for Economic Cooperation and Development (OECD) incorporated in NanoBridges software (29). The training molecules 14 and 18 were detected as an outlier and again best QSAR model (2) was modeled by deleting the outliers showing the best result on the SAH/MTAN inhibition. The developed QSAR model (2) can explain and predict 85.2% and 78.1% of variances of the SAH/MTAN inhibitory activity of the deliberated compounds. This model can also produce 68.5% external predictability and r2m (test) and SEE values of 0.636 and 0.490 respectively. This model is quite acceptable as per statistical validation. The square correlation coefficient between observed activities vs. predicted activities of the test compounds obtained from the correlation plot (Fig. 1) is calculated as 0.751, which suggests good model predictivity.
The model (2) parameters such as RDF55m, E1s, and AATSC7s have a positive impact on aromaticity toward SAH/MTAN inhibition, whereas the decrease in value of AATS1v and ATSC3s of the deliberated indazoles may increase the enzyme inhibition to stop the QS.
Indazole-SAH/MTAN docking
The resultant binding affinity along with details of amino acid residues bound with studied 40 indazole compounds toward inhibition of SAH/MTAN target is shown in Table IV. When ligands of interest are docked inside the defined target cavity of SAH/MTAN, both the conformational changes of ligand and receptor occur to make a number of ligand-receptor complexes. These ligand-receptor complexes represent the conformational rotation of the ligand inside the cavity. The ligand-receptor complex having a minimal dock score may produce maximum binding affinity toward the cavity of the target. The docking of indazole compounds having inhibition of SAH/MTAN resulted in common amino acid interaction inside the SAH/MTAN cavity for all compounds. These common amino acid residues such as ASP197, PHE151, GLU172, ILE152, and MET173 (highlighted in Tab. IV) are essential for ligand-protein interaction and help in unique conformational changing of ligand-receptor interaction complex, which is responsible for producing biological activity. If these amino acids are removed from the cavity then the binding affinity of a compound may be reduced. So these common amino acids play an important role in the suitable binding of the ligand toward the active site.
QSAR model-1 | |
pKi = 2.73333 (±0.84046) ‒3.13346 (±0.84654) CIC2 ‒0.96779 (±0.23832) nHBint4 +0.00324 (±0.00408) Am +1.06367 (±0.15668) C3SP2 ‒0.22577 (±0.07554) RDF135m. N = 29, R2 = 0.714, Q2 = 0.556, R2pred = 0.151, r2m (test) = 0.112, SEE = 0.660 |
|
Parameters | Physical interpretation |
CIC2 | Complementary Information Content index (neighborhood symmetry of 2-order) |
nHBint4 | Count of E-State descriptors of strength for potential Hydrogen Bonds of path length 4 |
Am | A total size index/weighted by mass |
C3SP2 | Doubly bound carbon bound to three other carbons |
RDF135m | Radial Distribution Function-135/weighted by mass |
QSAR model 2 | |
pKi = ‒2.66387 (±2.92509) ‒0.01691 (±0.00928) AATS1v +0.12995 (±0.01611) RDF55m + 9.69053 (±2.60499) E1s ‒0.0602 (±0.01124) ATSC3s +2.93253 (±0.59661) AATSC7s N = 27, R2 = 0.852, Q2 = 0.781, R2pred = 0.685, r2m (test) = 0.636, SEE = 0.490 |
|
Parameters | Physical interpretation |
AATS1v | Averaged Moreau Broto autocorrelation of lag 1 weighted by vdW volume |
RDF55m | Radial Distribution Function – 055/weighted by mass |
E1s | 1st component accessibility directional WHIM index/weighted by I-state |
ATSC3s | Centered Moreau Broto autocorrelation of lag 3 weighted by I-state |
AATSC7s | Averaged and centered Moreau Broto autocorrelation of lag 7 weighted by intrinsic state |
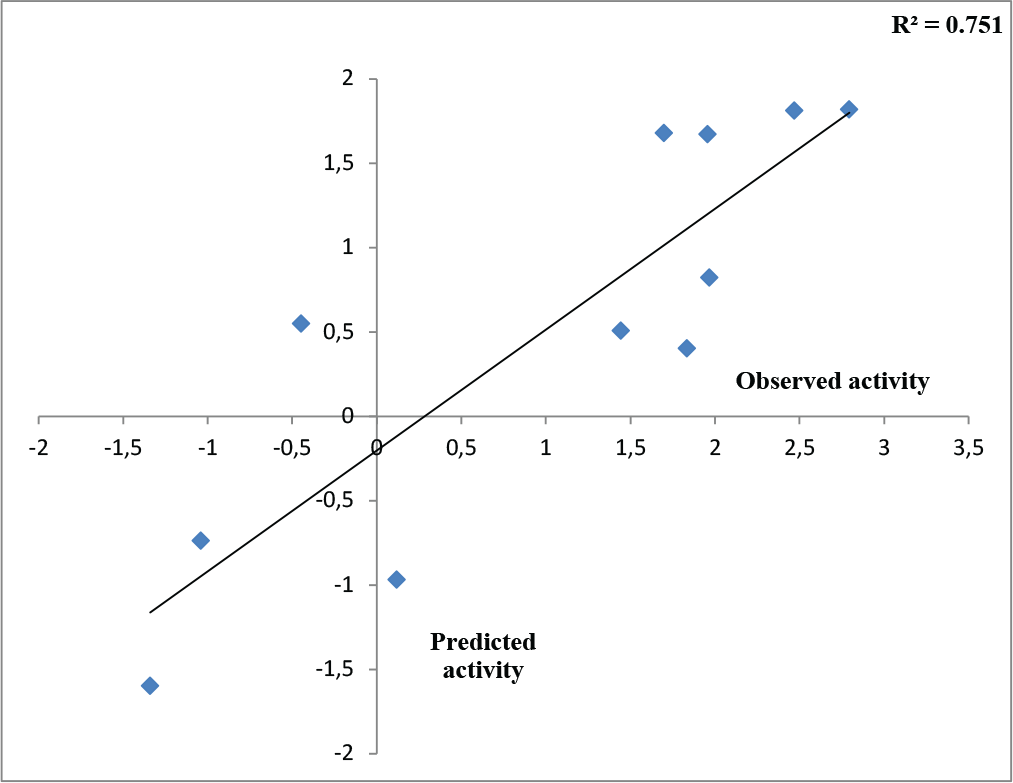
Fig. 1 - Observed versus predicted activity of test molecules after Outlier Analysis (model 2).
For a better interpretation of the binding mode of the indazole ligands, molecules are categorized into three highly active, intermediate active, and lower active by considering their biological activities (pKi) range as 1.50 to 2.79 µm, 0.44 to 1.4 µm, and <0.4 µm respectively. The highly active compounds such as 20, 22, 23, 26, 30-34, and 39 have more interactions with ALA150, PHE335, and VAL171 along with common amino acids bearing –14.477 to –11.191 kcal/mol dock score. The intermediate active compounds 24, 25, 27, 28, 35, 36, and 40 have –11.186 to –10.367 kcal/mol dock score with a lack of interactions with ALA150 except compound 28 which does not capture both ALA150 and PHE335. The remaining compounds are 1-18, 37, and 38 with lower active range having dock score greater than –10 kcal/mol, and these compounds lack either ALA150 and PHE335 or VAL171 amino acid interactions.
From Table IV, we can say that the binding affinity of compounds depends on a number of residues and minimal dock score involved in the ligand-receptor interactions. The highest active compound 21 shows the following pattern of interactions (Fig. 2).
Comp. no. | Amino acid residues interacting with ligand | pKi (µm) | Dock score |
---|---|---|---|
1. | ASP197, PHE151, GLU172, ILE152, MET173, ALA150, ALA199, GLY78, LEU158, VAL 171 | ‒0.447 | ‒10.2544 |
2. | ASP197, PHE151, GLU172, ILE152, MET173, ALA150, ALA77, GLY78, ASN153, SER196 | 0 | ‒10.134 |
3. | ASP197, PHE151, GLU172, ILE152, MET173, ALA150, GLY78, GLU 174, VAL171, LEU158 | ‒1.34 | ‒10.12 |
4. | ASP197, PHE151, GLU172, ILE152, MET173, ALA150, GLY78, SER76, ALA77 | ‒1.80 | ‒9.4128 |
5. | ASP197, PHE151, GLU172, ILE152, MET173, VAL 332, ALA150 | ‒1.43 | ‒9.4128 |
6. | ASP197, PHE151, GLU172, ILE152, MET173, PRO343, PHE335, VAL332, ALA334, ALA77, GLY78 | ‒1.23 | ‒10.931 |
7. | ASP197, PHE151, GLU172, ILE152, MET173, SER196, SER76, PHE335, VAL332, ALA150 | ‒0.71 | ‒9.49573 |
8. | ASP197, PHE151, GLU172, ILE152, MET173, ASN153, ALA150, VAL171 | ‒0.602 | ‒9.46457 |
9. | ASP197, PHE151, GLU172, ILE152, MET173, GLY78, SER76, ALA77, PHE335, ASN153, ALA150 | ‒1.041 | ‒10.4928 |
10. | ASP197, PHE151, GLU172, ILE152, MET173, HIS98, PRO343, ALA150, VAL171, ALA77, VAL332, GLY78, PHE335 | 0.119 | ‒9.88002 |
11. | ASP197, PHE151, GLU172, ILE152, MET173, ALA150, VAL332, ALA77, GLY78, ALA334, PHE335 | 0.301 | ‒10.631 |
12. | ASP197, PHE151, GLU172, ILE152, MET173, ALA199, LEU158, LEU80, GLY78, VAL171, ALA150, PHE335, GLU174 | ‒0.079 | ‒9.89744 |
13. | ASP197, PHE151, GLU172, ILE152, MET173, ALA150, VAL332, GLU174 | ‒0.431 | ‒9.78021 |
14. | ASP197, PHE151, GLU172, ILE152, MET173, GLY78, ALA77, VAL332, PHE335, ALA150 | ‒0.690 | ‒10.6611 |
15. | ASP197, PHE151, GLU172, ILE152, MET173, ALA150, VAL332, SER76 | ‒0.812 | ‒9.69068 |
16. | ASP197, PHE151, GLU172, ILE152, MET173, ALA150, PHE335, VAL332, GLU174 | ‒0.342 | ‒10.2657 |
17. | ASP197, PHE151, GLU172, ILE152, MET173, ALA150, PHE335, GLY78, ALA77, VAL171 | ‒0.255 | ‒11.4409 |
18. | ASP197, PHE151, GLU172, ILE152, MET173, ALA150, SER76, VAL332 | ‒0.361 | ‒10.6145 |
19. | ASP197, PHE151, GLU172, ILE152, MET173, ALA150, PHE335, PHE210, SER196, ALA334, VAL171 | 2.468 | ‒12.8469 |
20. | ASP197, PHE151, GLU172, ILE152, MET173, ALA150, PHE335, PHE210, SER196, ALA334, VAL171, SER76 | 1.903 | ‒12.855 |
21. | ASP197, PHE151, GLU172, ILE152, MET173, ALA150, PHE335, VAL171, GLY78, ALA77, ALA199, VAL332, TYR337, PRO343, LEU158 | 2.795 | ‒14.4772 |
22. | ASP197, PHE151, GLU172, ILE152, MET173, ALA150, PHE335, PHE210, SER196, VAL171, SER76 | 2.091 | ‒12.2961 |
23. | ASP197, PHE151, GLU172, ILE152, MET173, ALA150, VAL171, GLY78, ALA77, VAL332, PRO343, SER76 | 1.966 | ‒10.3176 |
24. | ASP197, PHE151, GLU172, ILE152, MET173, ALA150, PHE335, SER196, VAL171, GLY78, ALA77 | 1.443 | ‒10.367 |
25. | ASP197, PHE151, GLU172, ILE152, MET173, ALA150, PHE335, VAL171, GLY78, ALA77, VAL332, PHE207, ASN153 | 0.718 | ‒10.367 |
26. | ASP197, PHE151, GLU172, ILE152, MET173, ALA150, PHE335, SER196, VAL171, PHE201, SER76 | 1.545 | ‒11.1919 |
27. | ASP197, PHE151, GLU172, ILE152, MET173, PHE335, VAL171, GLY78, ALA77, VAL332, PRO343, SER76 | 1.283 | ‒11.0913 |
28. | ASP197, PHE151, GLU172, ILE152, MET173, VAL171, GLY78, ALA77 | 0.619 | ‒11.1815 |
29. | ASP197, PHE151, GLU172, ILE152, MET173, PHE335, VAL171, SER76 | 2.408 | ‒11.6766 |
30. | ASP197, PHE151, GLU172, ILE152, MET173, ALA150, PHE335, PHE210, SER196, VAL171, SER76 | 1.958 | ‒11.6479 |
31. | ASP197, PHE151, GLU172, ILE152, MET173, ALA150, PHE335, PHE210, LEU211, PRO343, GLY78, VAL332, ILE50 | 1.698 | ‒11.0191 |
32. | ASP197, PHE151, GLU172, ILE152, MET173, ALA150, PHE335, SER196, VAL171, VAL332 | 1.835 | ‒12.8532 |
33. | PHE151, GLU172, ILE152, MET173, ALA150, PHE335, PHE210, SER196, LEU158, VAL332, ASN153, GLY154 | 1.886 | ‒9.56766 |
34. | ASP197, PHE151, GLU172, ILE152, MET173, ALA150, SER196, VAL332, GLY78, SER76, ALA8, MET9, ALA77, GLU174, ILE50 | 1.769 | ‒12.697 |
35. | ASP197, PHE151, GLU172, ILE152, MET173, ALA150, PHE335, SER196, VAL171, VAL332, SER76, PRO343, PHE207, LEU211 | 0.443 | ‒12.8692 |
36. | ASP197, PHE151, GLU172, ILE152, MET173, PHE335, VAL171, VAL332, PHE210, GLY78 | 1 | ‒12.9969 |
37. | ASP197, PHE151, GLU172, ILE152, MET173, PHE335, VAL171, VAL332, SER76, PRO343, ALA77 | 0.026 | ‒14.2768 |
38. | ASP197, PHE151, GLU172, ILE152, MET173, ALA150, VAL171, VAL332, ASN153, GLY78, ALA77, PRO343, SER76 | 0.302 | ‒14.2777 |
39. | ASP197, PHE151, GLU172, ILE152, MET173, PHE335, VAL171 | 1.602 | ‒11.6493 |
40. | ASP197, PHE151, GLU172, ILE152, MET173, PHE335, PHE210, VAL332, GLY78 | 0.903 | ‒13.6849 |
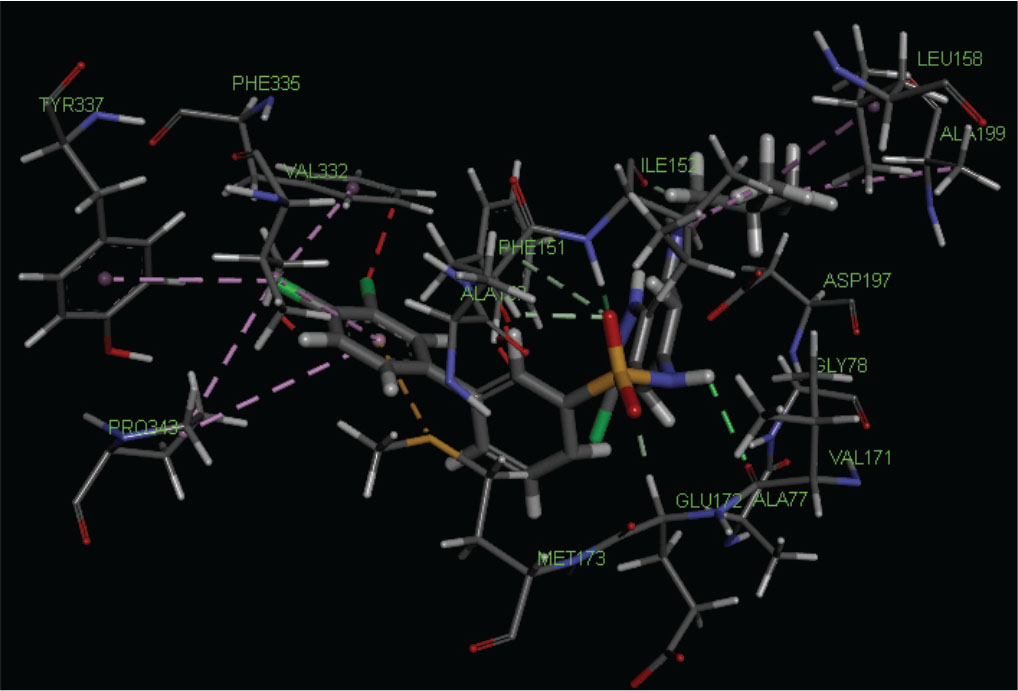
Fig. 2 - Best docking pose of highest active compound no. 21 docked in the cavity of S-adenosyl homocysteine/methylthioadenosine nucleosidase.
The amino group of sulfonyl amino linkage interacts with VAL171 and sulfonyl group interacts with GLU172, ILE152, and PHE151 by hydrogen bonding. ILE152 also interacts with isobutyl group by the same bonding. Chlorine atom at position-3 of 3,4-dichlorophenyl interacts with PHE335, TYR337, and PRO343, and VAL332 along with PRO343 interacts with 3,4-dichlorophenyl ring by hydrophobic bonding, whereas MET173 interacts with the sulfur bonding. Some amino acid residues such as ASP197, ALA150, ALA77, and GLY78 are also present at the binding site.
Conclusion
Parameters such as AATS1v, RDF55m, E1s, ATSC3s, and AATSC7s are crucially captured in the training QSAR model responsible for producing inhibition of SAH/MTAN. After molecular docking of indazole compounds, it was found that amino acids ASP197, PHE151, ILE152, GLU172, and MET173 are common for all compounds’ modes of interaction and to produce biological activity. Apart from that, the higher active compounds capture ALA150, PHE335, and VAL171 amino acid residues, which are very crucial for the inhibition of the SAH/MTA-mediated QS mechanism. The intermediate and lower active compounds lack any of these interactions. The binding affinity of indazole compounds depends on the number of amino acid residues involved in ligand-receptor interaction, for example, the highest active compound number 21 has 15 amino acid residues, which is more than the other 40 indazole compounds. A higher pIC50 value and minimal dock score help to find the greater binding affinity of the compound. This utility helps in lead optimization.
Disclosures
Conflict of interest: The authors declare no conflict of interest.
Financial support: This research received no specific grant from any funding agency in the public, commercial, or not-for-profit sectors.
Authors contribution: SN supervised RK and MK, who equally did the present work. AS contributed to the quorum sensing-mediated antimicrobial resistance.
References
- 1. Whiteley M, Diggle SP, Greenberg EP. Progress in and promise of bacterial quorum sensing research. Nature. 2017;551(7680):313-320. CrossRef PubMed
- 2. Jiang Q, Chen J, Yang C, Yin Y, Yao K. Quorum sensing: a prospective therapeutic target for bacterial diseases. BioMed Res Int. 2019;2019:2015978. CrossRef PubMed
- 3. Moré MI, Finger LD, Stryker JL, Fuqua C, Eberhard A, Winans SC. Enzymatic synthesis of a quorum-sensing autoinducer through use of defined substrates. Science. 1996;272(5268):1655-1658. CrossRef PubMed
- 4. Nandi S. Recent advances in ligand and structure based screening of potent quorum sensing inhibitors against antibiotic resistance induced bacterial virulence. Recent Pat Biotechnol. 2016;10(2):195-216. CrossRef PubMed
- 5. Parveen N, Cornell KA. Methylthioadenosine/S-adenosylhomocysteine nucleosidase, a critical enzyme for bacterial metabolism. Mol Microbiol. 2011;79(1):7-20. CrossRef PubMed
- 6. Kumar M, Saxena M, Saxena AK, Nandi S. Recent breakthroughs in various antimicrobial resistance induced quorum sensing biosynthetic pathway mediated targets and design of their inhibitors. Comb Chem High Throughput Screen. 2020;23(6):458-476. CrossRef PubMed
- 7. Schramm VL. Methods and compositions for treating bacterial infections by inhibiting quorum sensing. US20110190265;2011. Online
- 8. Tedder ME, Nie Z, Margosiak S, et al. Structure-based design, synthesis, and antimicrobial activity of purine derived SAH/MTA nucleosidase inhibitors. Bioorg Med Chem Lett. 2004;14(12):3165-3168. CrossRef PubMed
- 9. Li X, Chu S, Feher VA, et al. Structure-based design, synthesis, and antimicrobial activity of indazole-derived SAH/MTA nucleosidase inhibitors. J Med Chem. 2003;46(26):5663-5673. CrossRef PubMed
- 10. Halgren TA. Merck molecular force field. III. Molecular geometries and vibrational frequencies for MMFF94. J Comput Chem. 1996;17(5-6):553-586. CrossRef
- 11. Mills N. ChemDraw ultra 10.0. J Am Chem Soc. 2006;128(41):13649-13650. CrossRef
- 12. Yap CW. PaDEL-descriptor: an open source software to calculate molecular descriptors and fingerprints. J Comput Chem. 2011;32(7):1466-1474. CrossRef PubMed
- 13. Ballabio D, Consonni V, Mauri A, Claeys-Bruno M, Sergent M, Todeschini R. A novel variable reduction method adapted from space-filling designs. Chemom Intell Lab Syst. 2014;136:147-154. CrossRef
- 14. Hoffman BT, Kopajtic T, Katz JL, Newman AH. 2D QSAR modeling and preliminary database searching for dopamine transporter inhibitors using genetic algorithm variable selection of Molconn Z descriptors. J Med Chem. 2000;43(22):4151-4159. CrossRef PubMed
- 15. de Campos LJ, de Melo EB. Modeling structure-activity relationships of prodiginines with antimalarial activity using GA/MLR and OPS/PLS. J Mol Graph Model. 2014;54:19-31. CrossRef PubMed
- 16. Akaike H. Fitting autoregressive models for prediction. Ann Inst Stat Math. 1969;21(1):243-247. CrossRef
- 17. Ambure P, Aher RB, Gajewicz A, Puzyn T, Roy K. “NanoBRIDGES” software: open access tools to perform QSAR and nano-QSAR modeling. Chemom Intell Lab Syst. 2015;147:1-13. CrossRef
- 18. Golbraikh A, Tropsha A. Beware of q2! J Mol Graph Model. 2002;20(4):269-276. CrossRef PubMed
- 19. Roy K, Kar S, Ambure P. On a simple approach for determining applicability domain of QSAR models. Chemom Intell Lab Syst. 2015;145:22-29. CrossRef
- 20. Stahl M, Rarey M. Detailed analysis of scoring functions for virtual screening. J Med Chem. 2001;44(7):1035-1042. CrossRef PubMed
- 21. Nandi S, Bagchi MC. 3D-QSAR and molecular docking studies of 4-anilinoquinazoline derivatives: a rational approach to anticancer drug design. Mol Divers. 2010;14(1):27-38. CrossRef PubMed
- 22. Lee JE, Cornell KA, Riscoe MK, Howell PL. Structure of E. coli 5′-methylthioadenosine/S-adenosylhomocysteine nucleosidase reveals similarity to the purine nucleoside phosphorylases. Structure. 2001;9(10):941-953. CrossRef PubMed
- 23. Dey R, Nandi S, Samadder A. Pelargonidin mediated selective activation of p53 and parp proteins in preventing food additive induced genotoxicity: an in vivo coupled in silico molecular docking study. Eur J Pharm Sci. 2021;156:105586. CrossRef PubMed
- 24. Nandi S, Naaz A, Saxena M. Repurposing of potent Mtase inhibitors against ZIKV utilizing structure-based molecular docking. Int J Quant Struct Prop Relatsh. 2020;5(4):53-68. CrossRef
- 25. Nandi S, Kumar M, Saxena M, Saxena AK. The antiviral and antimalarial drug repurposing in quest of chemotherapeutics to combat COVID-19 utilizing structure-based molecular docking. Comb Chem High Throughput Screen. 2021;24(7):1055-1068. CrossRef PubMed
- 26. Thompson MA, Zerner MC. A theoretical examination of the electronic structure and spectroscopy of the photosynthetic reaction center from Rhodopseudomonas viridis. J Am Chem Soc. 1991;113(22):8210-8215. CrossRef
- 27. Golbraikh A, Tropsha A. Predictive QSAR modeling based on diversity sampling of experimental datasets for the training and test set selection. J Comput Aided Mol Des. 2002;16(5-6):357-369. CrossRef PubMed
- 28. Roy K, Mitra I, Kar S, Ojha PK, Das RN, Kabir H. Comparative studies on some metrics for external validation of QSPR models. J Chem Inf Model. 2012;52(2):396-408. CrossRef PubMed
- 29. Jaworska J, Nikolova-Jeliazkova N, Aldenberg T. QSAR applicability domain estimation by projection of the training set descriptor space: a review. Altern Lab Anim. 2005;33(5):445-459. CrossRef PubMed